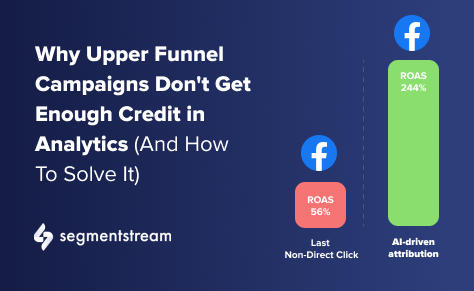
Why Upper Funnel Campaigns Don't Get Enough Credit In Analytics (And How To Solve It)
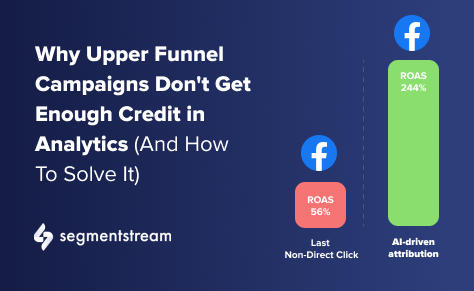
Even so the problem described in this article still remains the same, our approach has moved forward.
We still use machine learning to help future-thinking marketers to measure true value of their marketing channels, but in a much improved way.
Read about it in our announcement here.
Upper funnel campaigns are essential for any business to achieve its revenue goals.
Even though it’s enticing to run conversion campaigns to a cold audience, most of the time it’s just going to burn through the advertising budget.
Just like a salesperson asking a potential customer for a meeting in the first email probably won’t get great results (in fact, it might spark a negative reaction), asking a cold audience to buy your product won’t work very well.
In fact, in a lot of cases the person will not have heard of your brand or product before, so it’s essential to make people aware first before you ask them to buy.
Of course, most companies know this, so they run specific upper funnel campaigns which serve this exact purpose.
The attribution problem
According to research by Inc magazine, 98% of the website visitors don’t convert during the first visit.
Most businesses use Last-Click marketing attribution, which attributes the entire value of the conversion only to one channel - in most cases these will be the bottom funnel channels.
What will happen to upper funnel channels and campaigns is that in 98% of cases they will get zero value attributed.
And even if the business uses other multi-touch attribution models (i.e. Markov Chain or data-driven attribution), in most cases upper funnel campaigns will not receive accurate credit because all of these models work based on cookies which are not reliable when it comes to cross-device and cross-browser user journeys and are biased towards bottom funnel campaigns where the conversion happens.
Why is this a problem?
If the numbers tell you that a specific campaign is not profitable, it makes sense to pause it and put that marketing budget in other campaigns that do work.
This means that numbers would be telling you to take out nearly all the budget from upper funnel campaigns and put it into retargeting and other lower funnel campaigns.
However, it’s obvious your bottom funnel activities won’t work because they need the upper funnel campaigns to raise awareness first.
On the other hand, if you just continue to run upper funnel campaigns without paying attention to specific conversion numbers, you won’t be able to tell which campaigns work better than others and make improvements.
Retrospective vs predictive analytical approach
Most marketing attribution methods are based on cookies and are retrospective.
The main problem with retrospective attribution is that it’s technologically impossible to deterministically stitch user journey across multiple browsers and devices due to different cookies and the latest privacy regulations (ITP, GDPR, iOS tracking restriction).
For example, a user might click on an ad on Facebook, and Facebook mobile browser will open up. This browser has its own unique cookie, so if the customer will decide to make a purchase on another device or browser, for any marketing analytics system this will be a completely new user.
This means that any retrospective attribution models will not show you the proper picture of your marketing performance. Even using advanced multi-touch attribution models you will always see that your lower funnel channels and campaigns will perform a lot better compared to upper funnel campaigns.
This is why behaviour-based approach is much more accurate when analysing campaign and channel performance.
The True Value of Upper Funnel Campaigns
If we compare a Machine Learning approach to attribution versus the standard Last-Non-Direct-Click, we can see a massive difference in how many conversions each model attributes to each channel.
For instance, in the picture above it can be seen that the LNDC model attributes only 11 conversions to Facebook/cpc with a huge Cost of Sale of 53%.
If we only look at this data, one might conclude that social campaigns on Facebook are not working and the company is wasting a lot of money bringing 128,131 sessions to the website and getting only a few conversions.
However, according to Machine Learning, these Facebook campaigns are actually bringing in almost 237 conversions with the Cost of Sale as low as 2%. According to this, the marketing manager should invest even more in Facebook.
Why it is such a big difference? LNDC model only takes into account direct conversions where the user comes to the website and buys directly.
With ML model, a user might not buy right away, but the Machine Learning algorithm determines that based on the actions that the user took on the website (i.e. how much he/she engaged with the website), they have a specific % of probability to buy in the future (this probability can be anywhere 0-100% based on the ML).
So if a user comes from a social awareness campaign and then later buys from a retargeting campaign, LNDC will attribute the entire value of the conversion to the retargeting campaign while ML model will determine how much the user engaged with the website from the initial social campaign and proportionally distribute the value of the conversion across both campaigns.
How will the ML model determine how to distribute the value of the conversion between different touchpoints?
Unlike how it’s done with typical multi-touch attribution models (data-driven/exponential/linear), it is not determined by some fancy mathematical algorithm (i.e. Shapley value).
Rather, the conversion is distributed based on real website behavioural data that has been already collected. This means each business has a completely custom formula for multi-channel attribution assigned by its machine learning model.
This way, a marketing manager can see right away the true value of upper funnel campaigns and how much revenue they are driving to the business.
Find Out True Value of Your Upper Funnel Marketing
With SegmentStream’s platform you can see how much revenue each channel, campaign, ad and even single keyword has brought to your business - both upper and bottom funnel.
And this data is not based on some obscure mathematical algorithm - it comes directly from your own user behavioural data which is later analysed by machine learning.
Feel free to schedule a quick call with one of our team members and we will show you how this exciting technology works.
Optimal marketing
Achieve the most optimal marketing mix with SegmentStream
Talk to expert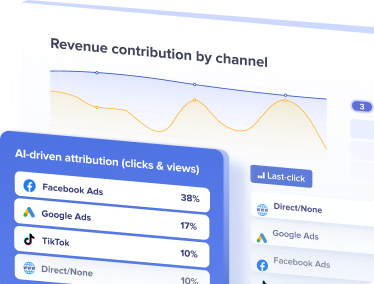