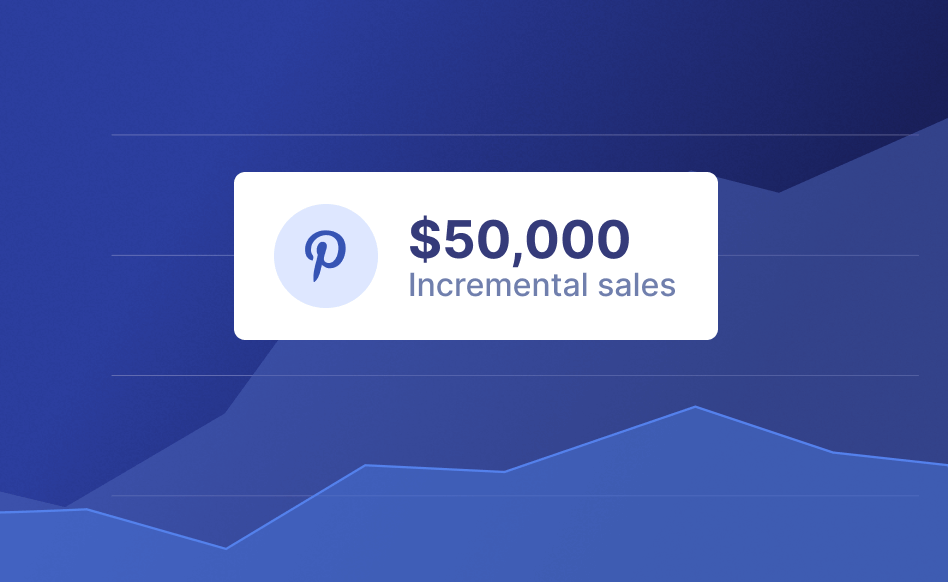
A guide to incrementality measurement: approaches, benefits and challenges
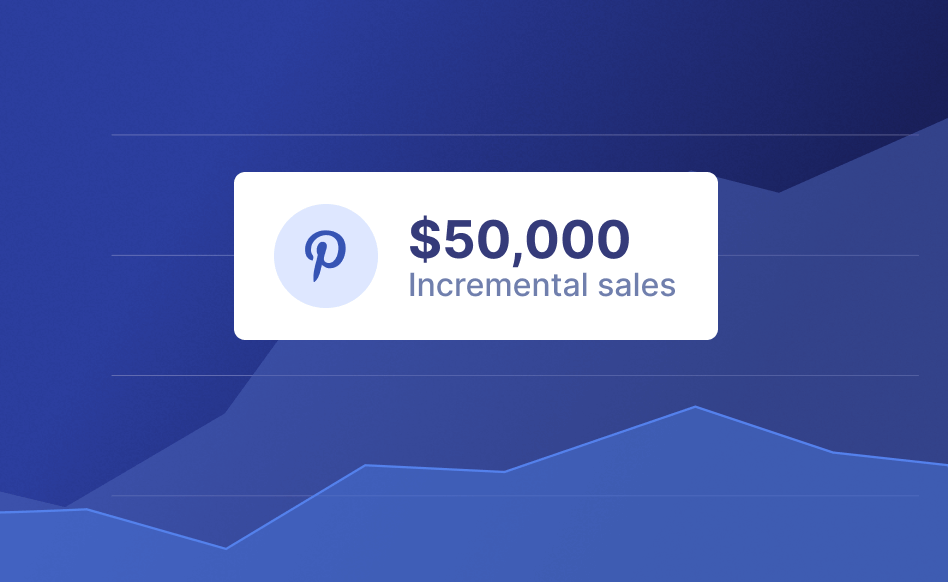
Incrementality measurement is essential for determining the real impact of your advertising efforts, offering a clear picture of how specific campaigns, tactics, or media channels contribute to sales growth. This guide explores the methodologies behind incrementality measurement and dives into the pros and cons of the approach.
How to measure incrementality?
Incrementality tests split your audience into two groups to understand the genuine effect of your ads:
- Test group: Exposed to advertising, measuring direct acquisition or remarketing impacts.
- Control group: Not exposed to the ads, serving as a baseline for comparison.
The difference in outcomes between these groups, expressed as incremental lift, quantifies the conversions directly attributable to advertising.
Incrementality testing example
Imagine a marketing team questioning the sales contribution from Pinterest ads. By pausing their Pinterest ad spend in select regions for 30 days, they aimed to discern the platform’s actual impact. The findings revealed Pinterest’s effectiveness was significantly higher than traditional attribution models suggested, affirming its value in driving sales.
Approaches to incrementality testing:
1. A/B testing
A/B testing is a straightforward method where you create two sets of campaigns or line items. One set displays public service announcements (PSAs) or “ghost ads,” while the other shows your standard creative ads. This setup aims to split the audience without control and compare the performance outcomes of both campaign sets, helping identify the incremental impact of your actual advertisements. The idea behind this A/B testing setup is to compare how audiences react to non-promotional content (PSAs or ghost ads) versus your actual marketing ads. By analyzing the difference in performance between these two sets, you can measure the incremental impact of your marketing efforts.
2. Budget holdout
Surprisingly simple yet profoundly effective, stopping all advertising for a period can sharply measure incrementality. This “dark period” establishes a baseline performance level, unimpacted by advertising efforts. Gradually reintroducing campaigns allows for monitoring the incremental effectiveness of each, providing insights into how advertising drives outcomes compared to the baseline.
3. Randomized control group
Similar to A/B testing and GEO split methods, using a randomized control group divides your audience into nearly identical segments: Group A and Group B. Each group is exposed to different ad types — PSA ads for control and campaign creatives for the test. This method aims to yield conclusive results by maintaining a controlled environment to measure the incremental effect accurately.
Benefits of Incrementality testing
- Cohort-level data ensures Incrementality testing remains reliable amidst user-level tracking restrictions, like those from iOS 17’s privacy update.
- Directly quantifies the sales impact of specific marketing efforts, such as a Facebook campaign, distinguishing its unique contribution.
- Delivers clear insights into the actual sales lift from targeted efforts without the bias present in traditional attribution models.
- Facilitates a test-and-learn approach for continuous budget allocation improvement, optimizing your marketing strategy.
Challenges in Incrementality testing
- Factors like seasonality, competition, or media mentions can drive user behaviour, necessitating careful consideration in measurement.
- Requires significant time and resources for proper setup and execution.
- While effective for evaluating specific tactics, it doesn’t offer the holistic view needed for long-term strategic planning.
- The typical duration of these tests complicates the timely assessment of new campaigns.
Incrementality testing vs Attribution modelling
Incrementality testing and Attribution modelling address two different aspects of marketing analysis, each essential for optimizing marketing strategies but serving distinct purposes.
- Incrementality testing evaluates a marketing campaign’s effectiveness by comparing a group exposed to the ads (test group) with a group that isn’t (control group). It aims to identify the sales directly generated by the campaign, distinguishing them from those that would have occurred without it.
- Attribution modelling: Instead of looking at direct impact, attribution modelling distributes credit for conversions across all touchpoints a customer encounters on their journey to conversion. It aims to paint a picture of how each channel contributes to the end goal.
Key differences of Incrementality testing vs Attribution
- Incrementality testing is about the ‘added effect’ of a campaign, focusing on what changes due to specific marketing actions. In contrast, attribution modelling seeks to understand and credit the entire conversion path, not just the impact of isolated actions.
- Incrementality testing thrives on iterative learning - a cycle of hypothesizing, executing tests, analyzing results, and refining strategies.
-
Traditional attribution models like data-driven for example attempt to account for the entire customer journey. However, given the cookie restrictions, ad blockers, and cross-device challenges, in 2024 tracking the full customer journey is impossible. Incrementality testing, on the other hand, is not so affected by modern tracking flaws.
Incrementality vs Marketing Mix Modelling
Marketing Mix Modeling (MMM) focuses on a holistic view by analyzing historical data to understand how various marketing elements (like media spend, promotions, and economic factors) collectively impact sales or other outcomes over time.
Key differences between Incrementality testing vs MMM
- Incrementality testing is designed to measure the additional value generated by a marketing action. MMM, on the other hand, offers a macro-level view, evaluating the cumulative effect of the entire marketing mix over a prolonged period.
- Incrementality testing adopts a test-and-learn approach, applying it to current campaigns to iteratively refine and enhance marketing strategies. This method, focusing on assessing the added impact of specific marketing actions, contrasts with Marketing Mix Modeling (MMM), which analyzes historical data for a broader, strategic overview of the marketing mix’s long-term effectiveness.
- Incrementality testing is ideal for tactical adjustments and optimizing current campaigns. In contrast, MMM is used for strategic decision-making, budget allocation, and forecasting the impact of marketing strategies over a longer period.
- MMM is particularly valuable for companies with significant marketing budgets and a diverse mix of channels, including TV and radio. In comparison, incrementality testing allows for more quicker, data-driven decisions without the need for extensive historical data.
How does SegmentStream measure incrementality?
SegmentStream adopts a budget holdout method, comparing marketing campaign performance in controlled markets against similar markets without those campaigns to measure true incremental impact.
How does it work?
Step 1: Choosing the right markets for an accurate incrementality test
Using a sophisticated engine, SegmentStream pinpoints control and test geo-regions that have similarities in revenue patterns and seasonality.
Step 2: Keep running ads in the control group, holding out in the test
Once regions are selected, the holdout phase begins. By disabling ad activity in the test group, SegmentStream measures which conversions would happen anyway, without ad activity.
Step 3: Analyzing and calculating the incremental uplift and ROAS
By comparing conversions and sales data between the test group and the control group, SegmentStream measures the true, incremental uplift generated by a particular ad channel or campaign.
There are multiple ways to run geo-incrementality tests: temporarily withholding ad spend in tested markets, or, on the contrary, launching new ads or doubling down on the budget in the test group while holding out in the control group.
SegmentStream’s methodology provides a robust framework for accurately measuring the incremental value of advertising efforts, empowering marketers to make informed decisions that enhance ROI and drive brand growth.
Interested to learn more? Book a meeting with our team!
Optimal marketing
Achieve the most optimal marketing mix with SegmentStream
Talk to expert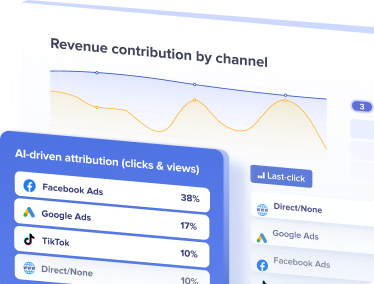