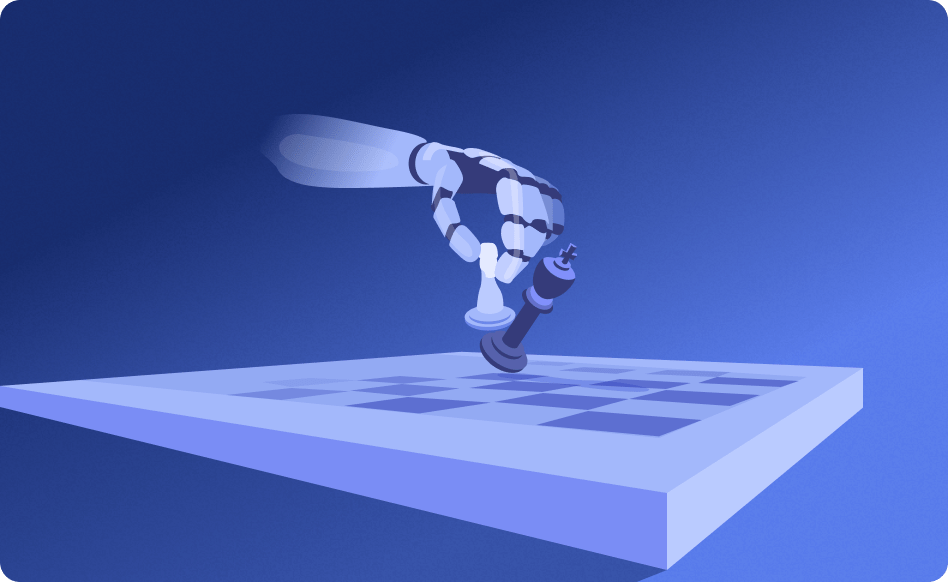
Future of marketing attribution: how AI changes the game
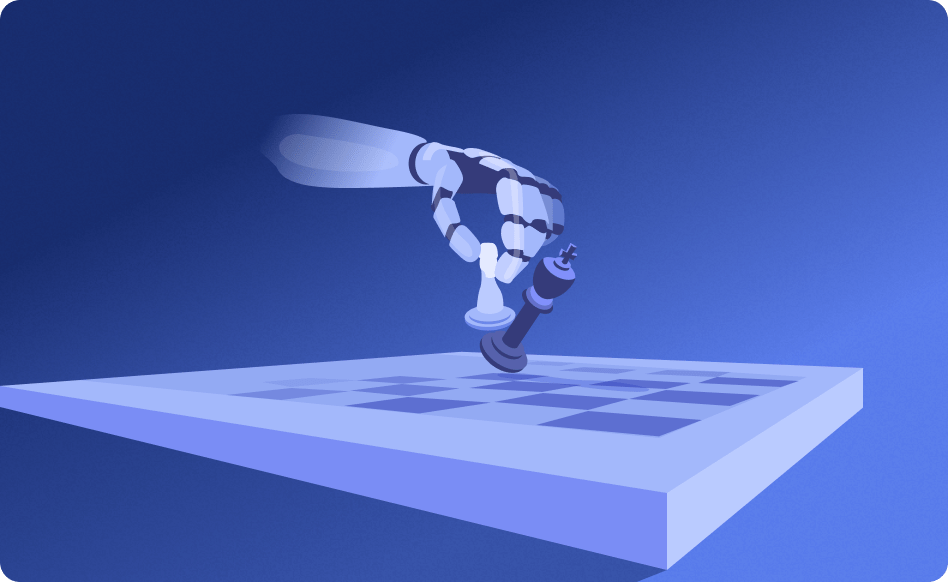
Traditional marketing attribution, based on rule-based methods, is struggling with today’s cookie restrictions and privacy regulations.
Now, Machine Learning and Artificial Intelligence have changed the game for marketing attribution. Let’s break it down.
Challenges in traditional attribution models
Traditional marketing attribution, based on rule-based methods, is struggling with today’s cookie restrictions and privacy regulations.
Now, Machine Learning and Artificial Intelligence have changed the game for marketing attribution. Let’s break it down.
Full trackability: a false promise
Multi-touch attribution’s key selling point, the ability to track every touchpoint in a customer’s journey, is proving unrealistic. The challenge of achieving a complete overview stems from several factors:
- Cross-device complexity: Consumers frequently switch between devices, complicating the tracking process.
- Privacy restrictions: Increasing privacy concerns and initiatives, like iOS 17’s Link Tracking Protection in Safari, limit tracking capabilities.
- Cookie limitations: Many browsers are moving towards restricting cookie use, impacting traditional tracking methods. Read how cookie restrictions impact attribution.
Heuristic-based models
Attribution models like “first-touch,” “last-touch,” and “U-shape” assign predefined values to touchpoints. However, these models are largely based on assumptions rather than empirical data, making their outcomes more speculative:
- Overlooking non-click Interactions: These models often ignore the impact of visual ads and social media impressions, which are crucial in shaping customer opinions but aren’t directly clickable.
Privacy and tracking vulnerabilities
Traditional models’ reliance on cookies makes them increasingly vulnerable:
- Evolving privacy standards: Regulations like GDPR and browser-specific privacy features are reducing the effectiveness of cookie-based tracking.
- Arising measures such as Apple’s iOS 17 update: The introduction of Link Tracking Protection has further complicated tracking, leading to fewer attributed conversions for major advertising platforms. Learn more
Cross-device/browser tracking Issues
The modern customer journey often involves multiple devices, from viewing ads on mobile to researching on desktop and purchasing on tablets. This cross-device behavior presents significant challenges for traditional attribution models.
Despite these challenges, the marketing world isn’t left in the dark. Alternative measurement approaches, less reliant on traditional tracking methods, are emerging and gaining traction in the industry.
Adapting to Modern Marketing Attribution with Machine Learning
Machine Learning (ML) and Artificial Intelligence (AI) offer solutions to these attribution challenges. They are particularly useful in a market where tracking customer journeys becomes complex due to multiple devices usage and privacy regulations.
Introducing ML-driven attribution
SegmentStream responds to these modern challenges by moving away from traditional cookie-based methods. Using ML and AI, it analyzes various data, including ad clicks and website user behavior, correlating this information with sales data in specific regions. This method helps in understanding the real impact of marketing channels and campaigns on overall revenue, even where direct cookie tracking isn’t viable.
Rule-based VS Machine Learning marketing attribution
The most adopted rule-based marketing attribution model is multi-touch attribution. It is supposed to divide the value from conversion between multiple touchpoints in the user journey that led to that conversion.
There are multiple types of multi-touch attribution:
- Linear attribution evenly distributes credit across all touchpoints that have contributed to a conversion.
- Time decay attribution gives more credit to touchpoints that are closer in time to the conversion than those closer to the beginning of the customer journey.
- U-shaped attribution gives equal weight to the first and last touchpoints in the customer journey while dividing the remaining credit equally among all other touchpoints.
- Data-driven attribution involves analysing data from various marketing channels to identify touchpoints that contributed to conversion and assigning weights to each touchpoint based on its contribution.
- Algorithmic attribution uses an algorithm to assign credit to each touchpoint based on factors such as the type of touchpoint, the position in the customer journey, and the time since the touchpoint occurred. This model requires a significant amount of data to train the algorithm.
Despite having a variety of multi-touch attribution models, marketers still have no real choice. Since there are many tracking restrictions and privacy regulations, even the most sophisticated multi-touch attribution tools show results similar to single-touch models.
On the other hand, there is ML-based marketing attribution. It is an innovative approach that solves all challenges that traditional attribution faces, in particular the lack of data due to tracking and cookie use regulations.
Advantages of ML-driven attribution
- SegmentStream looks beyond simple metrics, analyzing a range of user interactions for a fuller marketing picture.
- Shift from retrospective to predictive measurement. While traditional attribution models like multi-touch are trying to stitch the whole customer journey and wait for a conversion to happen to distribute its value across touchpoints it was able to track, SegmentStream analyzes impressions, behaviour and trends to provide real-time insights.
- SegmentStream uses a privacy-focused approach by moving away from user-based measurement to holistic analytics using advanced machine learning technologies.
- It goes beyond just clicks to provide view-through attribution. It allows to fairly evaluate upper-funnel activities like Social and Video campaigns.
- The New Marketing Mix Optimization solution helps marketers maximize ad campaign effectiveness with AI budget recommendations.
AI and ML technologies, as demonstrated by tools like SegmentStream, are becoming essential in modern marketing for accurate campaign analysis and optimization. These tools are key for businesses aiming to adapt and excel in today’s marketing environment.
To explore how SegmentStream can enhance your marketing attribution and optimization, contact us for more information.
Optimal marketing
Achieve the most optimal marketing mix with SegmentStream
Talk to expert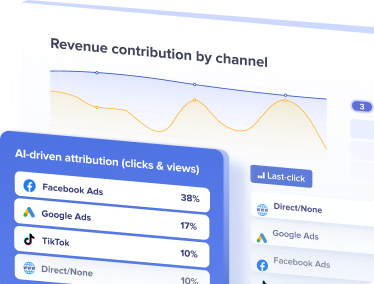