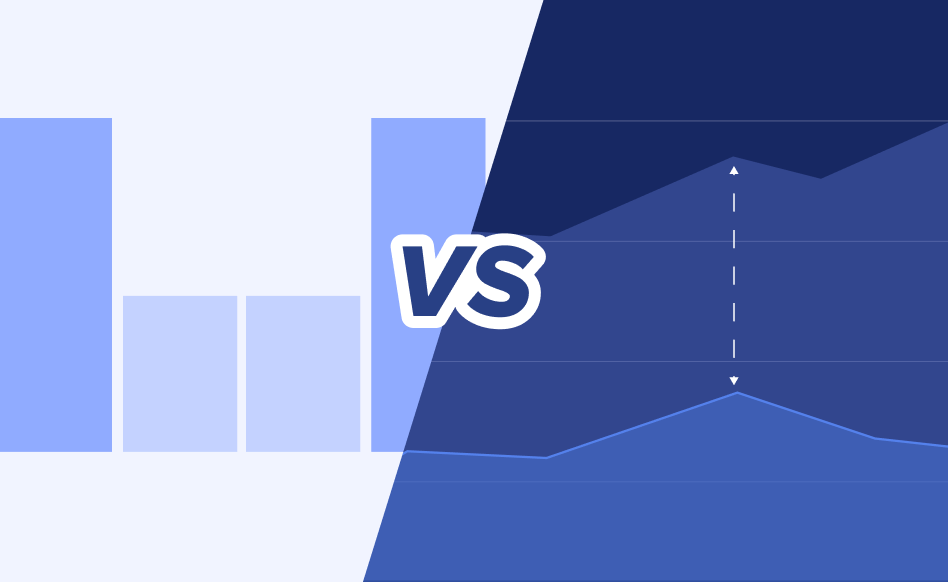
Incrementality testing vs Attribution modelling
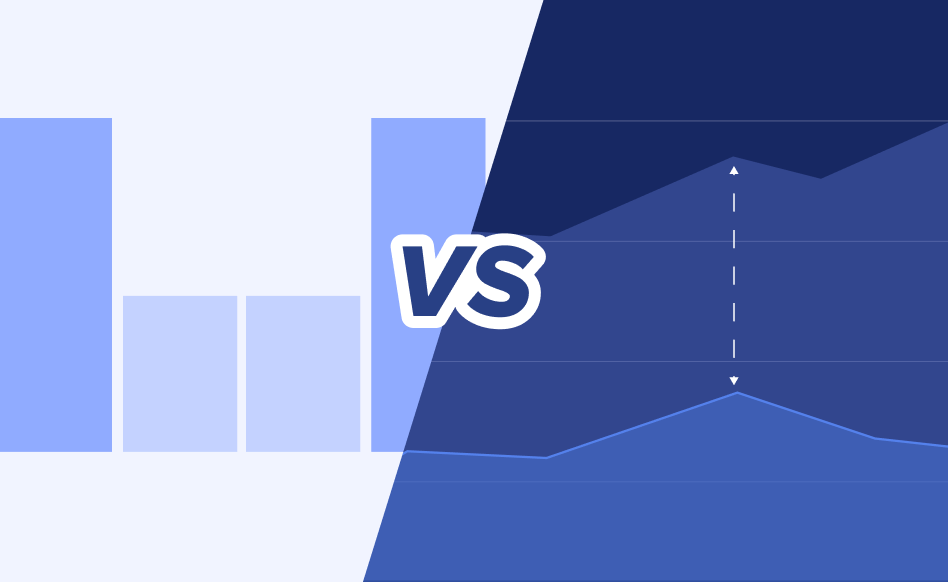
Incrementality testing
Incrementality testing measures the additional impact or ‘incremental lift’ that a marketing campaign contributes beyond what would naturally occur without it. This approach helps marketers understand the true effectiveness of their efforts by comparing sales from a group exposed to the campaign against a control group that was not. Whether you want to know how many new sales Facebook is driving, or the best media mix for your existing customers.
Benefits:
- It helps measure the impact of a particular marketing tactic or channel. For example, it can quantify the sales impact of a specific Facebook campaign, isolating its contribution from other marketing efforts.
- This method provides results without attribution bias, offering a clear view of the real sales lift generated by targeted efforts.
- You can use Incrementality testing for marketing mix optimization using a test-and-learn approach and continuously improve budget allocation.
Challenges:
- Setting up and running these tests requires significant time and resources, making them a substantial investment.
- While great for measuring specific campaigns, incrementality testing doesn’t provide holistic insights into the entire marketing mix, so it’s not suitable for long-term planning.
- It doesn’t help with evaluating freshly-based campaigns because normally the test lasts for around 30 days.
- Requires pausing other activities that could influence the test results, ensuring the accuracy of the incremental impact measurement.
Attribution modeling
Attribution modelling allocates conversion credit across various touchpoints in the customer journey, identifying influential channels and interactions leading to conversions.
Facing modern challenges like tracking restrictions and cookie regulations, traditional attribution methods such as last-click or multi-touch are increasingly unreliable.
In response, innovative, forward-looking solutions are emerging. For instance, SegmentStream’s AI-driven attribution. This model employs machine learning to sift through extensive data — from clicks and CRM data to website behaviour — to accurately gauge the contribution of each marketing effort to the bottom line.
Benefits of modern attribution solutions:
- Enables performance reporting across campaigns and channels by comparing key KPIs such as CPA and ROAS.
- Provides various attribution lenses, including advanced models that capture the value of visual channels often overlooked by click-only metrics. For example, SegmentStream introduces a nuanced perspective with its post-view and post-click attribution model. This method is especially valuable for evaluating visual-focused channels and campaigns, which traditional models, focused solely on clicks, often undervalue.
- Offers insights into newly launched campaigns, a capability absent in incrementality testing.
- Supports smart allocation decisions across the digital marketing mix, facilitating holistic optimization.
Challenges:
- Traditional models primarily focus on click-based metrics, systematically undervaluing channels like video and social media that significantly influence consumer decisions through visual engagement rather than direct clicks.
- Traditional attribution models heavily rely on tracking the whole customer journey using cookies. This makes them vulnerable to evolving privacy standards like GDPR, the fallout of private browsing and cookie restrictions in Safari, Mozilla, and soon Google. SegmentStream, on the other hand, surpasses those limitations by taking a different approach based on machine learning and 1st-party data.
- Approaches like multi-touch attribution are based on the false promise to track every touchpoint. But in reality, achieving a complete overview is impossible due to cross-browser and cross-device, tracking restrictions and long and complex user journeys.
- Attribution models like “first-touch,” “last-touch,” and “U-shape” assign fixed values to specific touchpoints. However, these assignments are based on assumptions, not concrete evidence, making them more guesswork than science.
Incrementality testing vs Attribution modelling: what to choose?
Incrementality testing evaluates specific marketing tactics or channels, testing hypotheses to guide tactical adjustments. It measures the added impact of a campaign by comparing results between a test group and a control group, helping optimize strategies based on incremental evidence.
On the other hand, Attribution modelling gives you a broader view, showing how different marketing activities contribute to conversions over time. This approach is great for day-to-day optimization and strategic planning, helping you understand the role of each channel in your marketing mix.
However, you don’t have to choose one over the other. Using both incrementality testing and attribution modelling together can significantly enhance your marketing strategies. Platforms like SegmentStream offer solutions that encompass both approaches. This approach enables you to make both quick tactical decisions and informed long-term budgeting choices, equipping you with a full suite of tools for effective marketing measurement and optimization.
Explore SegmentStream’s solutions for Attribution and Incrementality testing.
Optimal marketing
Achieve the most optimal marketing mix with SegmentStream
Talk to expert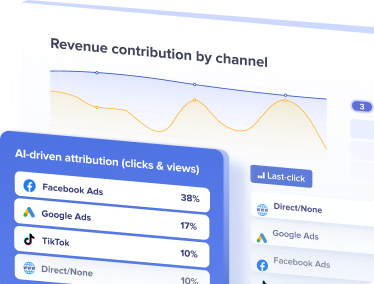