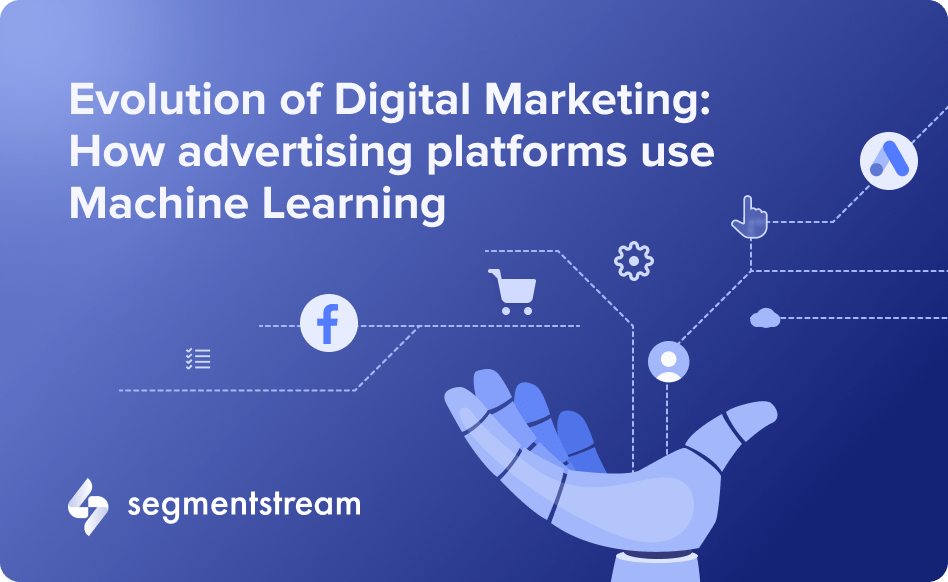
Evolution of digital marketing: How advertising platforms use Machine Learning
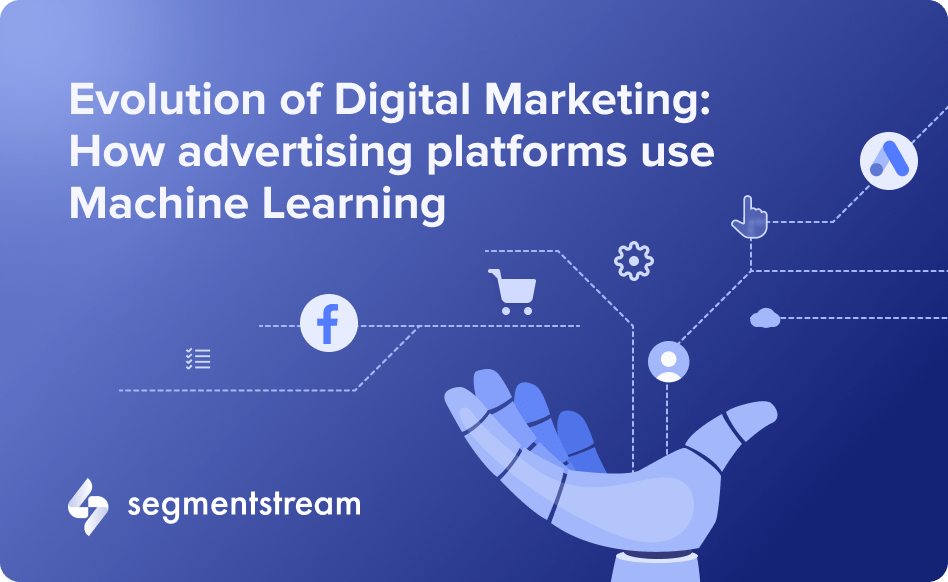
Machine Learning (ML) is a technology that is changing how advertising platforms operate forever. Now, ML is used in two major ways:
- ML algorithms are built-in to automate particular functionalities of advertising processes;
- ML-based marketing tools are used for better analysis and optimisation of advertising campaigns and marketing efforts.
In this article, we will dig deeper into the topic and see how exactly ML is revolutionising the advertising industry.
Digital marketing and paid advertising of today
Paid advertising is a huge part of marketing and probably the hardest one to measure. Despite having a plethora of tools out there, marketers still struggle with the analysis of results for ad campaigns as well as their future optimisation.
Take a look at this performance marketing loop:
This is how a typical digital advertising campaign is prepared:
- Marketers work on the content and prepare ads;
- They define the target audience;
- They set budgets;
- Then, the results are measured both during and after the campaigns.
The main problem here is that there are tons of combinations of budgets vs parameters of the target audience that make up thousands of combinations of ad campaigns themselves:
To deal with this extremely huge amount of ads, marketers use automation. The stages of defining target audiences and setting budgets are combined and are entrusted to auto-bidding strategies.
Auto-bidding tools are able to pick the best combinations of ads, audiences, and bids, but they need data to learn how to spot these successful combinations. These data are feedback signals — they indicate whether the ad was successful in motivating the user to convert.
Yet, automated bidding isn’t the only thing that ML is used for.
How Machine Learning works in ad platforms
When ML was first developed, it was predicted to reshape the future of ad platforms, and this is really happening. ML is primarily utilised for bidding automation but isn’t limited to it.
Machine Learning in Google Ads
Google uses ML algorithms for Smart Bidding — a feature that optimises bids for conversions or conversion value in real-time for every auction. Smart Bidding feeds on feedback signals — identifiable attributes of users such as device type, location, OS, etc., that suggest which users fit in the target audience best.
In bidding, ML algorithms train on available data to make an accurate prediction about how different bids impact conversions.
Another application of ML for Google Ads is Smart Creative. Here, the trained ML algorithm selects the best parts of creative assets to build the most successful combination for each customer.
Thanks to these ML-powered features, marketers can free up their time and focus on strategic planning.
Machine Learning in Facebook Ads
Facebook uses ML algorithms for similar purposes, for example, their feature Campaign Budget Optimisation is powered by ML. It allows for automatic budget management across ad sets in order to maximise the results for the lowest cost.
Also, Facebook Ads can optimise ad delivery automatically according to a set objective, e.g. conversion, link clicks, impressions, daily unique reach, etc.
Facebook Ads uses ML algorithms to deliver personalised ads to the right audience. Advertisers choose the preferred audience first, and then the platform gathers all ads that fit this audience and moves them to the auction stage.
How Machine Learning helps ad platforms deliver better results
What unites these two platforms is that in order to maximize the performance of ML algorithms inside automated bidding, it’s important to provide both platforms with feedback data on the value of each click that each ad set has received. The lack of feedback signals about this value prevents ML algorithms from efficient learning.
The whole issue then affects targeting and ad campaign optimisation — if these are managed poorly, this will lead to a massive loss in potential revenue.
Today’s complex user journeys are hard to observe and the conversion value cannot be distributed fairly between all traffic sources. This causes a lack of signals — most attribution tools will see that conversions fall into the “direct/none” category and feed this data to ML algorithms.
Sometimes there are just not enough signals for accurate learning — businesses that promote higher-value products and services can face the problem of “Limited Learning” when they don’t have the minimum number of conversions that the algorithms require to learn properly. Specifically, Facebook requires around 50 conversions per week, and Google needs 15 conversions within the last 30 days for Target ROAS campaigns.
And how can these issues be solved? Again, with ML.
How SegmentStream uses Machine Learning to tackle marketing attribution
SegmentStream applies Machine Learning (ML) to provide a sophisticated solution for marketing attribution, adapting to today’s digital marketing challenges. It analyzes extensive data, including user behavior and ad interactions, to identify effective marketing strategies. This approach surpasses traditional models by providing real-time insights and predictive analytics, crucial in a privacy-focused, cookie-restricted environment.
The platform measures the incremental impact of marketing campaigns and offers targeted budget optimization recommendations. It’s also designed to handle the complexities of cross-device consumer behaviors, ensuring a comprehensive understanding of the customer journey.
In essence, SegmentStream’s ML-driven approach offers a more accurate, privacy-compliant, and adaptable solution for modern marketing attribution challenges.
Optimal marketing
Achieve the most optimal marketing mix with SegmentStream
Talk to expert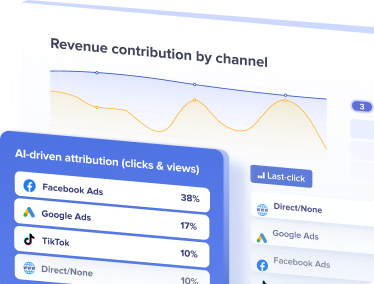