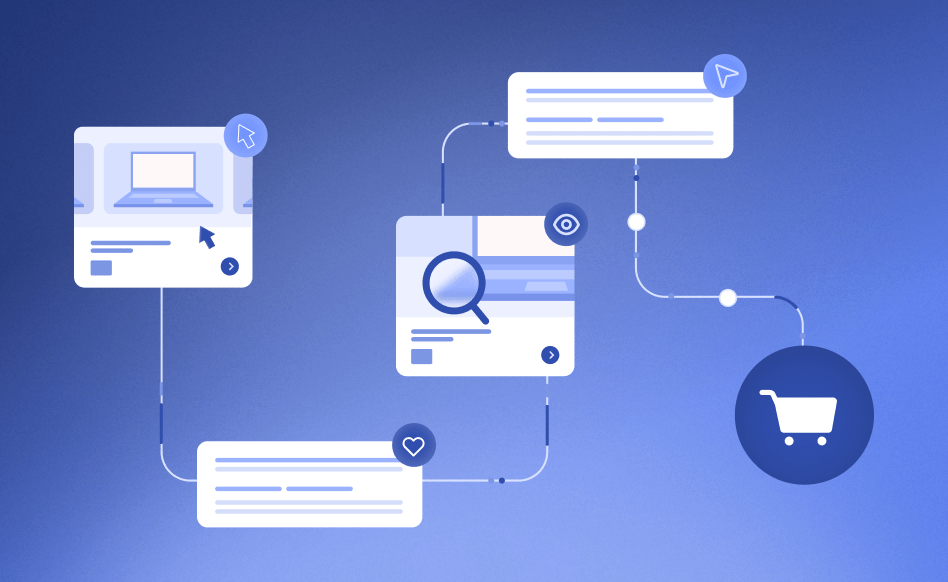
Marketing attribution is not what you probably think
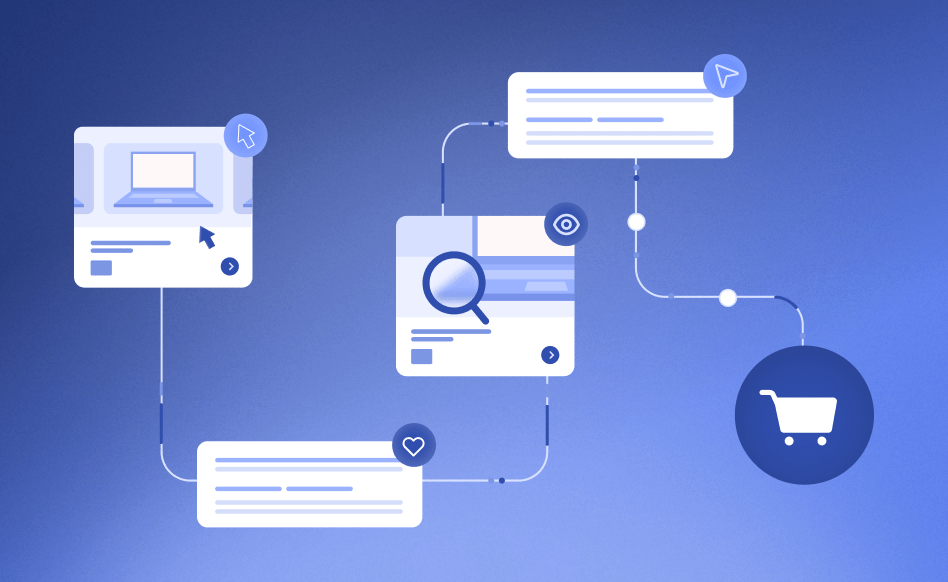
Marketing attribution is a crucial concept for every business, as it helps to understand which marketing efforts drive results and which don’t.
By analysing attribution data, marketers can make informed budget allocation decisions to achieve the highest return on their advertising investments. At the same time, advertising platforms such as Google Ads, Facebook Ads, and others use attribution data as feedback to improve their targeting and bidding algorithms.
Despite being such an important part of every business, attribution can still be a challenge for many marketers. The primary reason is often a misunderstanding of what marketing attribution actually is.
This article aims to resolve a typical misconception about what attribution modelling is and provide guidance on how to think about marketing attribution correctly.
What exactly is marketing attribution?
It is common to think that marketing attribution is the process of assigning credit from a conversion to the marketing channels and campaigns that led to that conversion.
However, that is not entirely true. This misconception often leads to wrong decisions and prevents businesses from achieving their primary goal, which is understanding the true value of their marketing traffic sources.
Here is a correct definition of marketing attribution, provided by Wikipedia:
But what is the difference, you may ask? To answer this question, let’s first explore how marketing attribution has evolved over time.
For a long time, marketing attribution models operated in the same way:
- A conversion happens. It could be a placed order on an e-commerce website, a website lead, a hotel booking, a phone call, or a closed deal — this varies from business to business. Let’s say the conversion is a purchase for $300.
- The user’s path to conversion is analysed. For example, before converting, the user interacted with the website three times: initially, they visited the website from a Google Ads campaign; then they returned from a retargeting display campaign; and finally, they converted from an email newsletter.
- Depending on the chosen attribution model, the business decides how to split the conversion value between these three touchpoints. For example, using the Last Click or last non-direct click attribution models, the entire value of the conversion ($300) would be assigned to the email channel.
But is it fair?
This is where the marketing attribution battles begin.
— Should email really receive all the credit? It doesn’t seem fair.
— Let’s give all the credit to Google Ads because it was the first channel!
— Hmm, that doesn’t sound fair either.
— What about giving each touchpoint equal credit then? $100 to Email, $100 to Retargeting, $100 to Google Ads?
— But why do we give equal credit? Maybe one channel was more valuable than the others.
— But what exactly does “more valuable” mean? How do we quantify this value?
— Let’s use algorithms!
In trying to find the right answer, marketers often go down the attribution rabbit hole, only to find out that no matter which model they choose, they are still not happy with the result.
In this search for the “holy grail,” some businesses have invested millions of dollars trying to build the best attribution model, only to find themselves returning to the good old Last Click model.
Why?
Well, the primary reason is that there was no significant difference in the results. What’s the point in using some complex attribution logic (That might even be hard to explain!) if the final marketing report still shows similar data to the simple Last Click model?
At this stage, some attribution experts start to give confident talks at conferences about how attribution doesn’t work anymore without even knowing what marketing attribution really means in the first place.
The biggest misconception of marketing attribution
Let’s recap the correct definition of marketing attribution provided by Wikipedia:
“The identification of a set of user actions that contribute to a desired outcome, and then the assignment of a value to each of these events.”
Now, let’s compare it with the common and misleading definition of attribution:
“The process of assigning credit from a conversion to the marketing channels and campaigns that led to this conversion.”
If you didn’t notice the difference, here’s a hint:
The biggest misconception of marketing attribution lies in thinking that a traffic source should receive value from a single conversion it contributed to.
This is the single biggest reason why many marketing attribution models fail to deliver. By trying to distribute the value from a single conversion, marketers and analysts become trapped in the idea of tracking customer journeys.
They want to know which traffic sources or touchpoints a user interacted with before converting and then distribute the value from this conversion accordingly. However, this approach can only work if you track the full customer journey from the very first touchpoint to the very last.
If the customer journey is not complete, then this whole approach falls apart. This is precisely what happens in most cases. No matter which attribution model is used, whether it is a simple one-touch model or a sophisticated data-driven model, neither can assign any value to traffic sources they simply can’t see.
It is not possible to track users’ real path to a conversion
One of the primary reasons — is cross-device and cross-browser interactions.
Today’s consumers often use multiple devices and browsers to access the web, making it challenging to track their activity across different platforms. This fragmentation makes it difficult to get a complete picture of a customer’s journey to conversion.
With the rise of in-app browsers of popular mobile apps, such as Facebook, Instagram, and Pinterest, this became an even more serious problem, as these in-app browsers contain a unique set of cookies. So in case, the person switches to their primary browser such as Safari or Chrome, the new customer journey will start, while the previous will end without a conversion.
The next challenge is the increasing prevalence of cookie restrictions.
Cookies are small pieces of data that are used to remember the user on the same website and track their activity across the web. However, many browsers are now blocking or deleting cookies, making it much more difficult to track online behaviour.
For example, Intelligent Tracking Prevention in Safari not only blocks third-party cookies, but also limits the lifespan of first-party cookies to 7 days, and in some cases, just to 1 day.
Short attribution windows are also a problem.
While the customer journey may be long and complex, the attribution window is limited. For example, the default attribution window of Facebook Ad Manager is a 7-day post click. This means that unless conversion happens within 7 days after the click, it will not be observed at all. This creates serious challenges for ad campaign optimisation, as conversion signals are an important feedback mechanism for ad targeting algorithms to learn.
Private browsing is another issue that affects marketing attribution.
Many users now use private browsing modes, which prevents cookies from being stored on their devices. This makes it impossible to track their activity across the web, making it more challenging to attribute conversions accurately.
As a result, marketing traffic sources do not receive fair value.
Initial traffic sources will not receive any credit for the conversion they contributed to.
Due to the challenges described above, the majority of website visits do not receive any value, even if they significantly contribute to conversions and revenue. In fact, up to 90% of conversions are not attributed to the initial traffic sources.
This creates two main problems for marketing teams:
1. Correct measurement and budget allocation are not possible due to the inability to track customer journeys.
All traditional marketing attribution models that distribute the value from a single conversion will fail to deliver. Even with First Click attribution, most upper-funnel campaigns are significantly undervalued.
This is the reason why most of the time marketers do not see much difference when comparing First Click attribution reports with the Last Click ones. In the image above, both with the First and Last Click, the entire value of the conversion will be attributed to a single traffic source - Direct/None.
2. Ad platforms struggle to target and optimise campaigns effectively.
Conversions are essential feedback mechanisms for Google and Facebook Ads, and other ad platforms. They rely on conversions to train their smart bidding algorithms and improve their targeting and optimisation capabilities. Providing ad platforms with more value signals as soon as possible is crucial for effective ad buying.
The problem is that due to broken customer journeys, the majority of clicks do not receive any value, as users rarely convert within a single website visit or cookie. It is especially challenging for businesses promoting high-value products or services, where buying journeys are longer and more complex. As a result, a lack of conversion signals prevents ad platforms from delivering desired results.
Due to incomplete and delayed feedback about the true value of each click, ad platforms such as Google and Facebook Ads are unable to deliver their best.
How to think about marketing attribution differently
If you have been asking yourself, “How to distribute the value from the conversion to the traffic sources that led to this conversion?”, you should start asking a different question.
The correct question is this: “How to distribute the total conversion value between the traffic sources according to their contribution to driving this value?”
By distributing the value from the total number of conversions, rather than a single conversion, you will guarantee that each traffic source gets a proper value, even if the actual conversion does not happen within the same cookie. For example:
- The advertising campaign generated a website visit on mobile.
- The user did not convert during the initial website visit.
- After some time, the user returns to the website from the desktop and makes a purchase.
Here, the initial advertising campaign is responsible for driving a sale, even though it will not be directly attributed to the campaign by any attribution model that relies on tracking the user’s path to conversion using cookies. However, it is clear that both website visits contributed to the purchase and, as a result, should both receive value.
The question now is: How to measure this contribution?
How to measure the contribution of each traffic source
People cannot make a purchase without visiting your website and going through a set of steps to complete the conversion.
Before buying, users will do their research, visit multiple pages on the website, view product images, read FAQs, and take other actions before making a final purchasing decision. Therefore, every website visit and every action taken has an incremental effect on driving a user towards a goal — whether it is a purchase, booking, or lead form completion.
By analysing user behaviour patterns, you can identify which set of actions lead to the conversion, and which don’t. This data, when analysed properly, will provide you with valuable insights into how different website visits contribute to sales.
Here is a simple example:
- The first user visited your website but left after a few seconds without converting.
- At the same time, there might be another user who also left the website without purchasing, but unlike the first one, they’ve spent 15 minutes browsing through the website, carefully choosing the product they are interested in, and returning to it multiple times.
Are these two non-converting website visits equal?
Of course not. The chances that the second user will complete a purchase are much higher than in the first case.
However, what you will see in your analytics tool is that two non-converting visits have the same value, as they did not result in directly attributed conversions. Ad platforms that drive these users to the website will also receive the same information and will be unable to target more high-quality users with a higher likelihood to purchase.
Now, imagine that you could assign value to these two non-converting visits depending on the information you have. Most likely, you will assign far more value to a more engaged website visit. Even if you assign a value using synthetic metrics (i.e., 1 point to ‘Visit A’ and 5 points to ‘Visit B’), it will still help both you and your ad platforms understand which traffic sources drive more valuable users to your website with the highest potential to convert in the future.
However, this approach is not entirely reliable, especially at scale. The primary problem is that real user behaviour patterns are much more complex than in our example above, and they cannot be described using a simple rule-based formula.
Even if the user spent at least 2 minutes on the website, it doesn’t necessarily mean that they are actually moving towards the purchase. Many additional factors and nuances need to be considered, which the human brain cannot process manually. These factors include information about the user, the context of the website visit, product pricing if you are selling multiple products, and many other data points.
As a result, when you have thousands of website visits, you cannot make correct conclusions about how valuable each visit is using a gut feeling that this particular website visit is more valuable than another.
To find correlations between the enormous amount of information and actual conversions, it can be helpful to use the power of cloud computing and Artificial Intelligence.
How to assign proper value to each visit using Machine Learning
SegmentStream relies on first-party data and machine learning to give a fuller picture of how paid media contributes to sales, moving past traditional methods that depend on cookies.
SegmentStream finds a correlation between ad spend, clicks, website visits (incl. user behaviour) and total sales in the same region/country.
This way, SegmentStream measures true contribution of each channel and campaign on total revenue, even when conversions cannot be directly attributed using cookies.
1. Automated Marketing Reporting to measure key metrics in one place.
2. Cookieless Attribution. It analyzes ad clicks, impressions, costs and user behaviour to measure the incremental impact of ad campaigns. Learn more here.
3. Marketing Mix Optimization. The platform provides AI-driven budget recommendations to achieve an optimised marketing mix. Learn more here.
To sum up
Attribution, despite being one of the most important things in digital marketing, is often misunderstood. Relying on the common knowledge that digital marketing attribution is responsible for assigning a value from a single conversion to the traffic sources that led to this conversion creates many challenges in understanding the true impact of digital marketing sources.
Marketing sources that contribute to conversions and revenue often simply do not get proper credit due to tracking restrictions and complex customer journeys. Therefore, any model that relies on tracking a user’s path to conversion will fail to deliver accurate insights and will be misleading for the marketing teams in their budget reallocation process.
This creates the same challenge for popular ad platforms as well since they rely on accurate attribution data to train and tune their automated targeting and bidding algorithms.
Therefore, it is crucial to shift the focus from a single conversion to the total conversions that the business has generated across all traffic sources combined. Then, this value should be distributed between traffic sources accordingly to their incremental contribution to driving sales, even if these sales cannot be attributed directly.
There are multiple ways to achieve this. However, one of the easiest and most reliable ways to understand the contribution of each website visit is to use AI-driven attribution solutions that don’t rely solely on cookies, such as SegmentStream.
This is an innovative measurement approach that relies on using a combination of first-party data and Machine Learning to assign value to each website visit based on its impact on driving predicted conversions, rather than past conversions.
This approach eliminates the challenges of traditional, retrospective attribution models as it guarantees that each website visit and traffic source will get the accurate value even if the real conversion happens from another device, browser, or cookie.
Use SegmentStream to gain visibility into the real value of your traffic sources, as well as unlock the full potential of your ad campaigns.
To learn more about SegmentStream and its capabilities book a demo with one of our solution consultants.
Optimal marketing
Achieve the most optimal marketing mix with SegmentStream
Talk to expert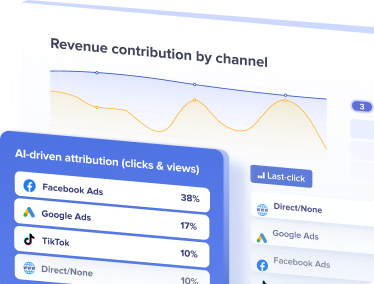