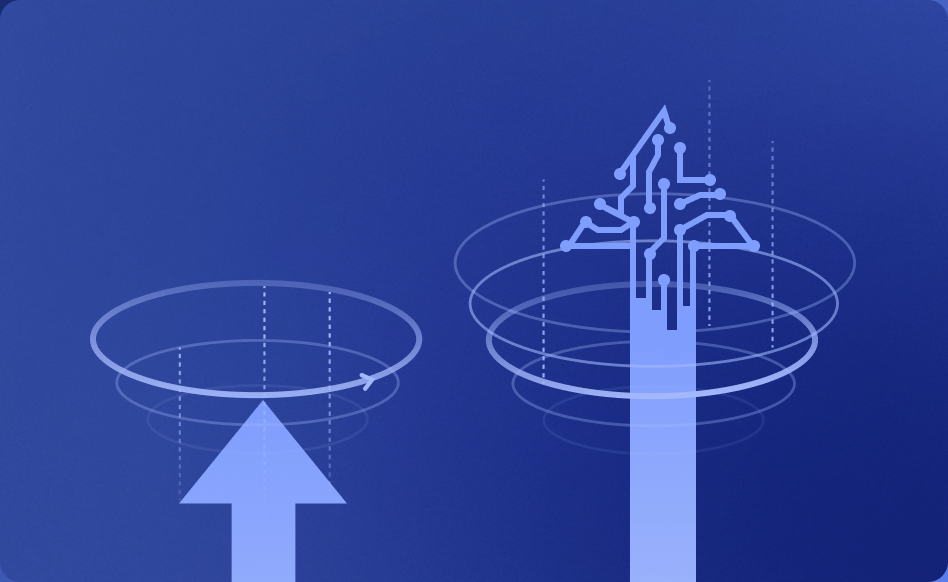
Predictive vs Data-Driven marketing attribution
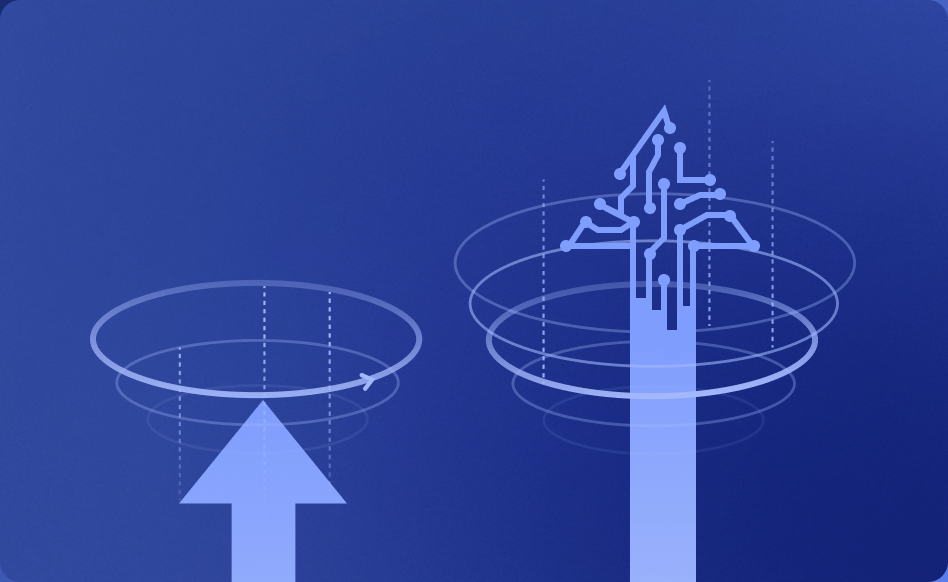
The difference between data-driven and predictive attribution models is that the former struggles in the cookie-less era, while the latter, with predictive technologies on the rise, is gaining traction.
Let’s explore why traditional data-driven models: the Markov chains and The Shapley Value are no longer viable and what are the benefits of a predictive approach.
What is data-driven attribution?
Data-driven attribution evaluates the entire customer journey, determining the impact of each touchpoint on conversion likelihood. It compares paths leading to conversions against those that don’t, crediting touchpoints based on their conversion influence.
Types of data-driven attribution models
The most popular are:
- Markov chains: It uses historical data to determine how likely a customer is to move from one touchpoint to the next, assigning credit based on these probabilities.
- Shapley value: Based on game theory, this model evaluates the average impact of each touchpoint on a sale, considering all possible touchpoint combinations.
Challenges of data-driven attribution
In theory, it should allow for a more accurate and dynamic understanding of each touchpoint’s effectiveness in the conversion process.
In reality, it struggles with:
- Cross-device journeys.
- Long sales cycles and short attribution windows.
- Tracking restrictions and cookie regulations.
That’s why it’s safe to say that data-driven attribution is built on a false promise of full traceability, which in a cookieless world is no longer possible.
Predictive attribution vs. data-driven attribution: what’s the difference?
The main distinction lies in their approach: data-driven attribution is retrospective: it looks at past data to assign credit to marketing channels. Predictive attribution, on the other hand, uses current data and trends to anticipate future outcomes, adapting to changing marketing conditions.
What is predictive attribution?
Predictive attribution uses statistical models and machine learning to identify which marketing actions are likely to result in a conversion. It analyzes user behaviour, a key advantage in the cookieless world, where the focus is shifting from detailed user-based analytics to broader, privacy-centric approaches.
For example, it involves ‘what-if’ scenarios. These help predict what might happen if, for example, you spend more on ads in a particular channel or change your target audience.
SegmentStream’s predictive attribution and optimization solution
SegmentStream employs advanced AI to analyze the connection between digital advertising and specific revenue outcomes in distinct regions or cities.
It relies on first-party data and machine learning to give a fuller picture of how much paid media contributes to sales, moving past traditional methods that depend on cookies.
It offers two main solutions:
1. AI-driven attribution:
SegmentStream analyzes user behaviour to measure incremental ROAS across digital campaigns without relying solely on cookies. Learn more here.
2. AI-driven Marketing Mix Optimization:
The platform provides AI-driven budget recommendations to achieve an optimized marketing mix. Learn more here.
Conclusion: DDA or predictive attribution?
Data-driven attribution, once the standard for marketing analytics, is now limited by privacy regulations and the complexity of tracking across devices. Predictive attribution emerges as a more fitting choice for several reasons:
- Effective in a cookieless world: Predictive attribution doesn’t solely depend on cookies. It focuses on analyzing user behaviour trends, transitioning from user-based analytics to an AI-driven approach that fits better with current privacy standards.
- It doesn’t wait for a conversion to happen. Instead, predictive attribution forecasts possible conversions, providing valuable campaign insights proactively. Which is especially useful for evaluating freshly launched campaigns or for companies with long sales cycles.
- It’s more advanced: Predictive attribution looks at a wide array of data points, from clicks, impressions, and costs on ad platforms to user interactions on websites.
Read our success stories to learn how major companies like L’Oréal, KitchenAid and Oxford Summer Course use predictive technology to better analyze and optimize their marketing mix.
Optimal marketing
Achieve the most optimal marketing mix with SegmentStream
Request a demo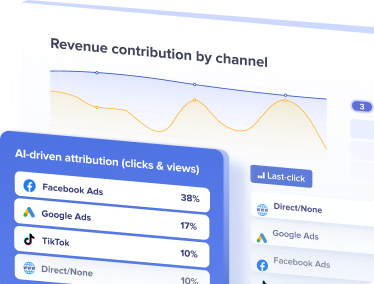