Complete Guide to Digital Marketing Analytics Solutions
Marketing measurement 101 — learn about the most adopted approaches to marketing analytics. Find out how they work with cookies, cross-device and cross-browser journeys.
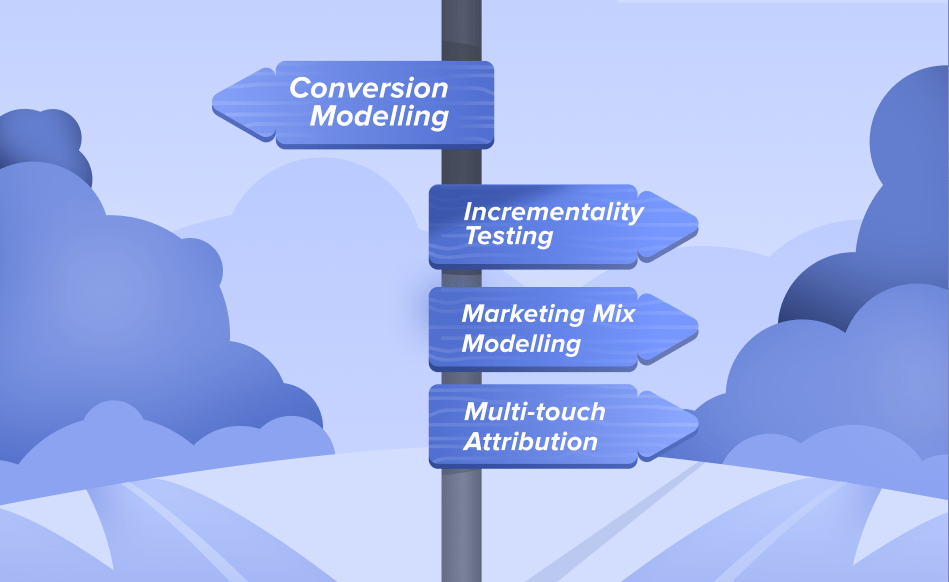
What is this guide about?
When choosing between available options, such as Multi-Touch Attribution, Marketing Mix Modelling, etc. marketers need to decide which approach will work best for their business.
However, the majority of modern marketing analytics solutions are dramatically affected by cookie restrictions and tracking limitations, hence can no longer provide adequate results.
So how to pick the right one? This guide will help you make the right choice.
Read this guide to find out how these approaches compare to each other, their pros and cons, how they compete with Conversion Modelling, and how to utilise them to get the most out of each one. This digital marketing guide has been crafted to become every performance marketer’s handbook of marketing analytics tools.
In this guide you’ll learn:
- Digital marketing approaches: an overview
- Multi-Touch Attribution: pros and cons
- Marketing Mix Modelling: pros and cons
- Incrementality Testing: pros and cons
- Conversions Modelling: pros and cons
- How to choose wisely from all analytics solutions?
- How SegmentStream can help
Digital Marketing Approaches at a Glance
Let’s have a quick look at data-driven marketing solutions we will be reviewing in our guide.
Multi-Touch Attribution
Multi-Touch Attribution (MTA) evolved from the single-touch attribution commonly used by most of measurement tools. Its main goal is to assess the value of the marketing channels within the user’s path to conversion.
While single-touch attribution models like Last Click, Last Non-Direct Click, and First Click will assign 100% of the conversion credit to one channel within the path, Multi-Touch Attribution determines the value of every touch point on the user’s way to a conversion. The most common MTA approaches are Time Decay, U-Shape, Linear and Data-Driven.
MTA requires all touch points to have a common identifier like a cookie or user ID, that would serve as a joining key to stitch those touch points into a single path.
Example: Performance marketing and analytics teams are willing to explore the user journey and understand the impact of each touchpoint within it. They turned to Data-Driven Attribution to assess how their channel and campaign mix performs. With it, they are able to analyse the path to conversion that happens only within a single cookie.
Marketing Mix Modelling
Marketing Mix Modelling (MMM) is a thorough statistical analysis of all sales over a long period of time, from a couple of months to several years. It is often regression-based and also covers the factors that have probably caused the sales. MMM looks over budget allocations to different marketing channels, both online and offline, on a macro level — these are campaigns on TV and radio, digital marketing activities, sponsored events, and so on.
It takes into account how competitors behaved over the analysed period, what the market conditions were, as well as seasonality and other factors. Then, MMM will look for any spikes in sales and examine whether they were caused by the aforementioned factors or not, and calculate the impact of future campaigns and activities.
Example: A large company that produces everyday consumer goods wants to revise all their marketing activities. The Chief Marketing Officer (CMO) turns to MMM once a year to gain insights into sales and make further budget allocation decisions in a data-driven way.
Incrementality Testing
Incrementality Testing is basically A/B testing that measures the incremental value of marketing strategies and tactics. The main difference here is that Incremental testing will divide the target audience into two groups — test and control. The test group will be exposed to those marketing activities which impact you need to measure, and the control group remains unexposed.
Then, after the experiment is finished, you compare the performance of two groups and calculate the conversion difference between the groups — this is how you get the incrementality. What you’ll see is the percentage of conversions that happened thanks to the tested media exposure beyond those that would have happened anyway.
The Incrementality Testing tools are typically divided into two separate groups: cookie-based and cookieless tools. Tools that are used to assess the efficiency of digital ads will rely on cookies to split the audience and calculate the results. These tests are called auction-split and audience-split, which means that the audience is split at different moments — either when the auction happens or when test and control groups are targeting different audiences based on cookies.
The cookieless solutions split the audience by geographical or demographical parameters, time, or products. They also take into account such metrics as revenue, new site visitors, CPA, and other metrics that traditional A/B tests would typically assess as well.
Example: CMO wants to examine whether Facebook ads are worth spending budget on — they see low performance based on the last non-direct click attribution. One way to test this would be to stop the ads for some time and observe whether the amount of conversions changes. However, this is a risky decision for any business. To evaluate the performance of the ads in a safer way, the CMO launches Incrementality Testing and exposes the test group to Facebook ads while the control group remains unexposed.
When they compare the results of both groups they notice that the test group generated $190K in sales revenue and the control one generated only $100k. Thus, the sales for $100K would have happened anyway and Facebook Ads generated an additional $90K which is 47% incremental sales revenue. Therefore, CMO considers Facebook Ads a decent channel and decides to run the campaigns further.
Conversion Modelling
Conversion Modelling uses Machine Learning (ML) to assess the impact of each website visit and calculate the conversion probability based on visitor’s behaviour data. The approach works even when the actual conversion cannot be observed because of cookie limitations and tracking restrictions.
You can use Conversion Modelling output to make budget allocation decisions across all your channels, including upper-funnel activities, and perform digital ad optimisation through sending more valuable signals to ad platforms and leveraging them in the smart bidding strategies.
Example: Performance marketers and analysts always want to know the true value of their marketing activities, especially paid ones. They use Conversion Modelling daily to gain real-time insights into campaign performance and make wise budget allocation decisions. These insights are also used for smart-bidding optimisation within ad platforms — modelled conversions serve as feedback signals.
Multi-Touch Attribution: Pros and Cons
Pros:
- If a common join key, like a Cookie ID or a User ID, is present across multiple touchpoints, MTA allows users to analyse the customer journey within this single ID.
- This approach aims to help with a broad range of use cases from strategic and tactical media planning to budget allocation and ads optimisation.
Cons:
- Since MTA heavily relies on cookies, it is not able to account for cross-device and cross-browser users behavior. It could have worked perfectly if customers used only one device and browser, but this is far away from the modern reality.
- For the same single cookie reason, this solution is impacted by ITP limitations which shorten the life of a cookie down to 7 days or even 1 day if we talk about paid-media clicks. This makes MTA not suitable for sales cycles that are longer than 1-7 days.
Marketing Mix Modelling: Pros and Cons
Pros:
- MMM provides a fundamental approach to assess all your marketing activities, including digital, TV and offline advertising, like billboards and flyers, events, sponsorships, etc. It takes a wide range of different external factors into account, like seasonality, inventory levels, market conditions, competition, and other. This makes it a great tool to take strategic level budget allocation decisions that go beyond digitals marketing.
- This tool is not tied to cookies and is not impacted by ITP and other cookie limitations as it typically operates on a macro channel level.
Cons:
- MMM requires tons of data to perform the analysis. You might need from several months to multiple years of data to build a healthy well-rounded model.
* Since it is used on a macro level only, it doesn’t allow granular data deep dive beyond high channel level such as “TV”, “Billboards”, etc. There are tools in the market that run MMM for digital ads only, however the channel would still be the lowest level of granularity you can get, i.e. “direct”, “paid-search”, “paid-social”.
* Cannot be used for freshly launched campaigns as it requires quite a lot of time to build the model and assess the results.
Incrementality Testing: Pros and Cons
Cookie-based Incrementality Testing: Pros and Cons
Pros:
- Auction-based and audience-based tools can ensure a proper random split of audiences, which guarantees that the test is fair.
- Incrementality Testing tools can help on both strategic and tactical levels with media planning and also assist in making decisions about budget reallocation even on an ad group level.
* The tools don’t require collecting historical data prior to launching the test itself, so it is that simple — just set up the experiment and wait for the data to collect.
Cons:
- These tools require the conversions to happen within one cookie to be able to split the audience properly. Otherwise, the results won’t be assigned to the test and control groups accordingly. Now, observing complete paths to conversion is nearly impossible due to cross-browser and cross-device journeys and tracking limitations. Therefore, these tools don’t get enough conversion data and become biased towards lower-funnel activities.
- To assess the results of Incrementality Testing, there should be a significant amount of captured conversions — just like Multi-Touch Attribution requires. Typically, it takes around 1 to 30 days to capture conversions depending on the attribution window of your business. Since there are restrictions that limit the life of a cookie down to 1 to 7 days, like ITP, audience-based and auction-based Incrementality Testing tools will work properly only if your customers convert within the same cookie, which means within 1 to 7 days after the initial click.
* The results of incrementality testing can be used for manual budget adjustments, but the data can’t be sent to ad platforms for automatic ad optimisation.
Cookieless Incrementality Testing: Pros and Cons
Pros:
- Cookieless tools don’t depend on cookies and just split the audience by other parameters: geographical, demographical, etc. The conversion can be captured even if the customer converts via a different device or browser as long as they’re in a test or control group.
* These tools aren’t impacted by ITP limitations, tracking restrictions, or short attribution windows, which is good for longer sales cycles.
* No historical data is needed here as well.
Cons:
- The audience splits used here are less random, if compared to ones performed by cookie-based tools. There are too many additional factors that can impact both test and control groups such as sale periods and local holidays, or just other online ad channels.
- To compare the incrementality of different channels, you need to run several tests and make sure that each time the control group is kept outside of the tested channel. This way, the ability to attract new users and scale the business is limited.
* It is usually extremely hard to run experiments for specific channels in isolation, and almost impossible to pinpoint particular campaigns. And we’re not even speaking about ad groups here! Therefore, using them for tactical planning and optimisation while going beyond the channel level is close to impossible. If done, it’s still impossible to observe the impact of optimisation activities.
Conversion Modelling: Pros and Cons
Pros:
- Conversion Modelling provides you with a holistic overview of all channels that can also be broken down into campaigns, ad groups, ads, and even keyword levels. It’s easy to compare the performance of channels and tactics, and the results can be used to make wiser budget allocation decisions on both strategic and tactical levels.
Once you have a stable ML model inside the Conversion Modelling Platform, you can start taking action based on the results of the analysis immediately. The tool works even for freshly-launched campaigns.
Conversion Modelling can tackle cookie restrictions and tracking limitations because it evaluates the value of each visit right after it happens. It calculates the probability to convert and doesn’t wait for the actual conversion to happen. Therefore, this approach is well aligned with cross-device and cross-browser journeys and long sales cycles.
Cons:
- The approach requires at least 3 weeks of historical data for the ML model to be trained.
- The model requires data about at least 300 online conversions monthly to ensure good prediction scores.
How to Choose an Optimal Analytical Solution?
All four solutions serve different purposes and have different requirements and limitations. When you choose the ones that would work your business you should always start with defining the challenge you’re trying to solve. Also, remember that each tool can become a valuable asset for your business if applied correctly, so we suggest using them together for a synergetic effect.
If you need a fundamental overview of all marketing activities then consider choosing Marketing Mix Modelling. It will help you to assess all marketing channels, both digital and offline. Yet, note that this tool can’t be used for freshly launched campaigns and on-the-go adjustments — the tool is very high-level and can only do retrospective analysis.
Incrementality Testing is another good option, but leveraging this tool as a testing mechanism requires extra attention. You’ll have to keep the control group completely unexposed to the tested campaign or channel, which is hard. Also, this limits your ability to scale as well as leads to lots of missed opportunities and lost revenue.
If you want to get a deeper understanding of your users’ paths to conversion and use obtained insights for better optimisation of ads, you could try MTA. Just remember that due to modern cookie tracking restrictions it is no longer possible to see the entire user journey. It diminishes the value of MTA tools, and might lead to incorrect insights about the value of each traffic source.
And if you’re tired of dealing with ITP and complex customer journeys, we recommend trying Conversion Modelling Platform. This tool is a new way to assess your Marketing Mix, get more signals for your ads, and boost performance measurement and optimisation.
If you’d like to learn more about how SegmentStream can drive better results for your Performance Max campaigns, feel free to request a demo of Conversion Modelling Platform.
Optimal marketing
Achieve the most optimal marketing mix with SegmentStream
Talk to expert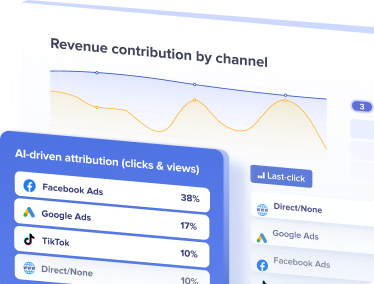