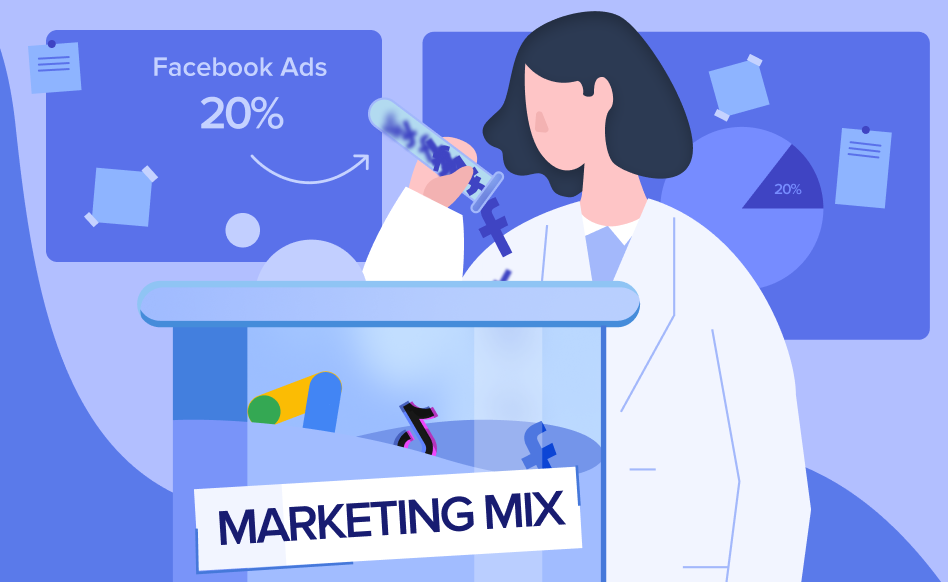
Marketing mix optimisation: solutions, tools and challenges
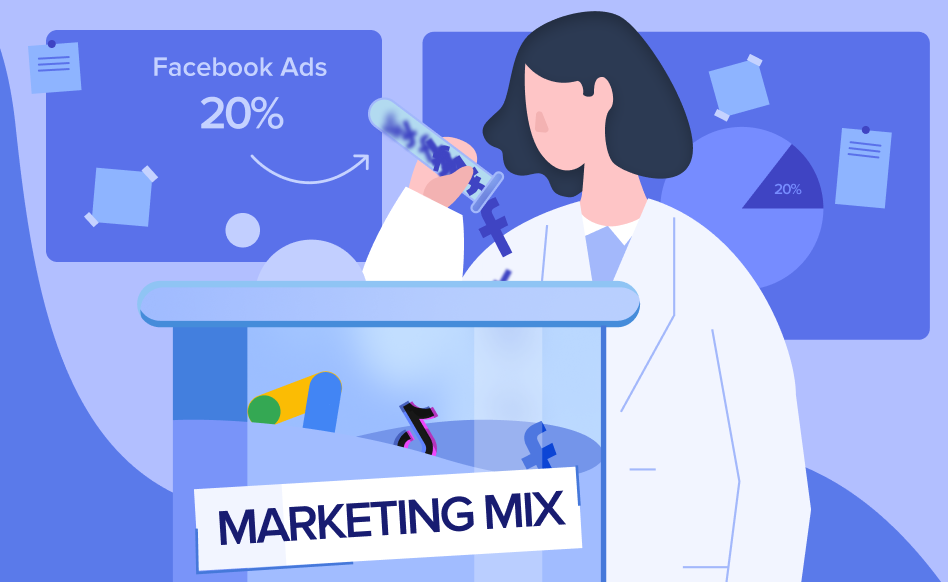
Disclaimer: The focus of this article is on broad marketing strategy optimization by smartly distributing your budget across different channels.
Ad platform bias
Conversion data from ad platforms is often unreliable because each platform has its way of attributing sales. For example, if a user clicks on a Facebook ad but later makes a purchase after clicking a Google ad, both platforms might claim credit for the sale. This overlap shows why you can’t fully trust the conversion numbers reported by ad platforms.
Measurement сhallenge
Advanced measurement approaches include attribution modelling, marketing mix modelling (MMM), and incrementality testing, each facing distinct challenges:
1. Attribution
If you’ve successfully sidestepped challenge #1 by adopting a marketing analytics platform to unify your data for cross-channel performance analysis, you’ll then need to choose one or more attribution models to accurately assign credit to the appropriate channels and campaigns.
However, attribution poses significant challenges for advertisers for several reasons:
- Traditional tools are retrospective, meaning they only assign value to touchpoints after a conversion has occurred. This method falls short for products or services with long consideration phases, as many interactions may be overlooked due to brief attribution windows or cookie deletions. For instance, consider the impact of Safari’s Intelligent Tracking Prevention (ITP).
- The attempt to piece together complex customer journeys which is impossible.
-
Restrictions such as GDPR, privacy laws, and cookie limitations complicate tracking efforts. Further details are available here.
- Attribution models often rely on speculative logic, assigning predefined values to certain touchpoints based on assumptions rather than empirical evidence. This makes the process more akin to guesswork than scientific reasoning.
- The focus on click-based interactions means that channels primarily visual in nature, like video and social media, are undervalued. Despite their significant influence on consumer behavior, these channels are often overlooked because traditional attribution models do not account for non-click interactions.
For a more in-depth exploration of these attribution challenges, you can read further here.
2. Incrementality testing
Incrementality testing uses a test-and-learn approach for ad budget optimization, starting with a hypothesis about ad impact.
For instance, the audience is divided into two groups: one that is shown an ad and another that isn’t. The objective is to determine the incremental contribution by measuring the sales difference between these groups.
Optimizing ad budget through incrementality testing:
Incrementality testing allows teams to strategically adjust their ad spend based on the effectiveness of specific channels. For instance, a marketing team might suspect that TikTok ads significantly impact sales but aren’t fully recognized by traditional attribution methods. After conducting an incremental lift test, they found that the group exposed to TikTok ads resulted in 3x more sales than the non-exposed group. It prompts them to allocate more budget to TikTok.
Incrementality testing can range from straightforward audience split tests, like the one mentioned, to more complex geo-scale tests when spending in a specific region is increased to observe the effect, similar to exploring “what if” scenarios in Marketing Mix Modeling (MMM).
Challenges with incrementality testing:
- The test-and-learn method is time-consuming and demands considerable effort.
- Newly launched campaigns are hard to evaluate quickly due to the time required for testing.
- It doesn’t allow for a holistic marketing mix evaluation for precise media budget planning for a quarter or year, as MMM does.
- Deciding what to test requires formulating specific hypotheses.
- To conduct tests in-house you would need a dedicated team.
- To get buy-in for the experiments you have to explain to your team the test design, audience division, and significance of results etc.
3. MMM (Marketing Mix Modeling)
MMM software helps in the holistic budget allocation across media channels. This approach, rooted in the 1950s, has evolved significantly. Today, leading companies like SegmentStream, Pecan, and Northbeam have updated MMM with machine learning, offering marketers advanced tools for smarter budget distribution. Explore MMM further.
Challenges of MMM:
- Understanding its complexity: The leap into modern MMM requires belief in its effectiveness due to its sophisticated, machine learning-driven methodology. It promises a cookieless and optimal marketing mix, however, the technology is complex, making it challenging for those without a data science background to fully understand how it works.
- Cost: Be prepared for substantial investment, as MMM solutions can cost up to $100,000 per year.
- It’s not for every company: MMM is particularly beneficial for companies with significant digital advertising expenditures, typically more than $1 million per month.
Summary
Choosing the right data for budget decisions is crucial in marketing mix optimization. We’ve identified key challenges within the most advanced methods today:
- Attribution modelling is evolving from its traditional, backwards-looking approach, thanks to AI-driven enhancements by companies like SegmentStream, offering improved accuracy for tracking customer journeys in a cookieless world.
- Incrementality Testing appeals to those who favour a test-and-learn methodology. While it provides valuable insights into the incremental impact of specific marketing actions, it’s important to recognize its limitations: the tests require significant time to conduct and fail to offer immediate insights, making it a less agile approach.
- Marketing Mix Modeling (MMM) is undergoing a renaissance, thanks to machine learning technologies from innovators like SegmentStream, Pecan, and Nexoya. Designed for large-scale advertisers with monthly digital ad spends above $1 million, MMM delivers detailed insights into the marketing mix, though it comes at a higher cost.
Optimal marketing
Achieve the most optimal marketing mix with SegmentStream
Talk to expert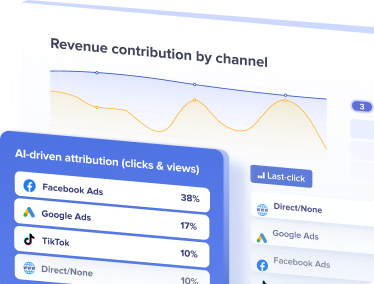