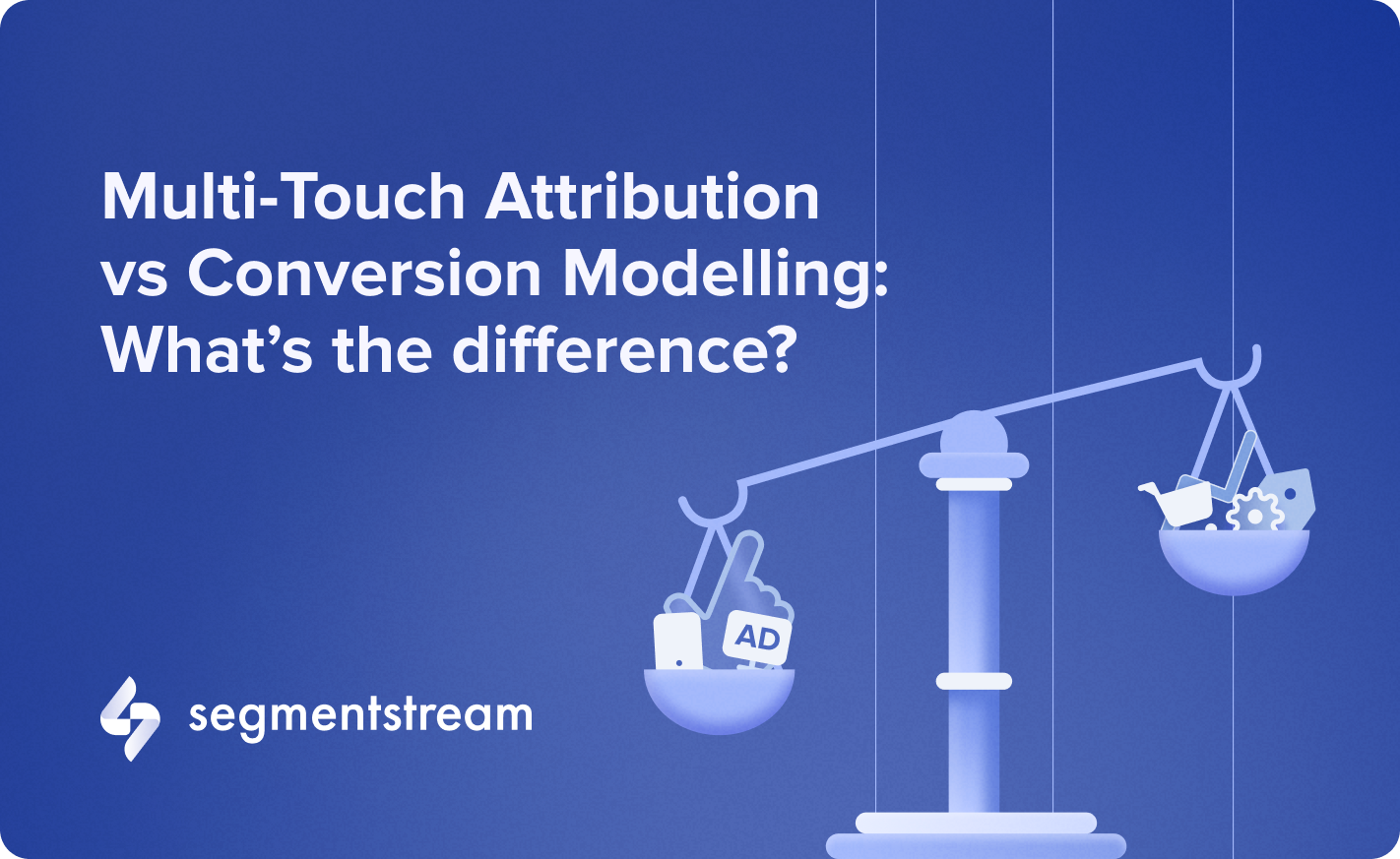
Multi-Touch Attribution vs SegmentStream: What’s the difference?
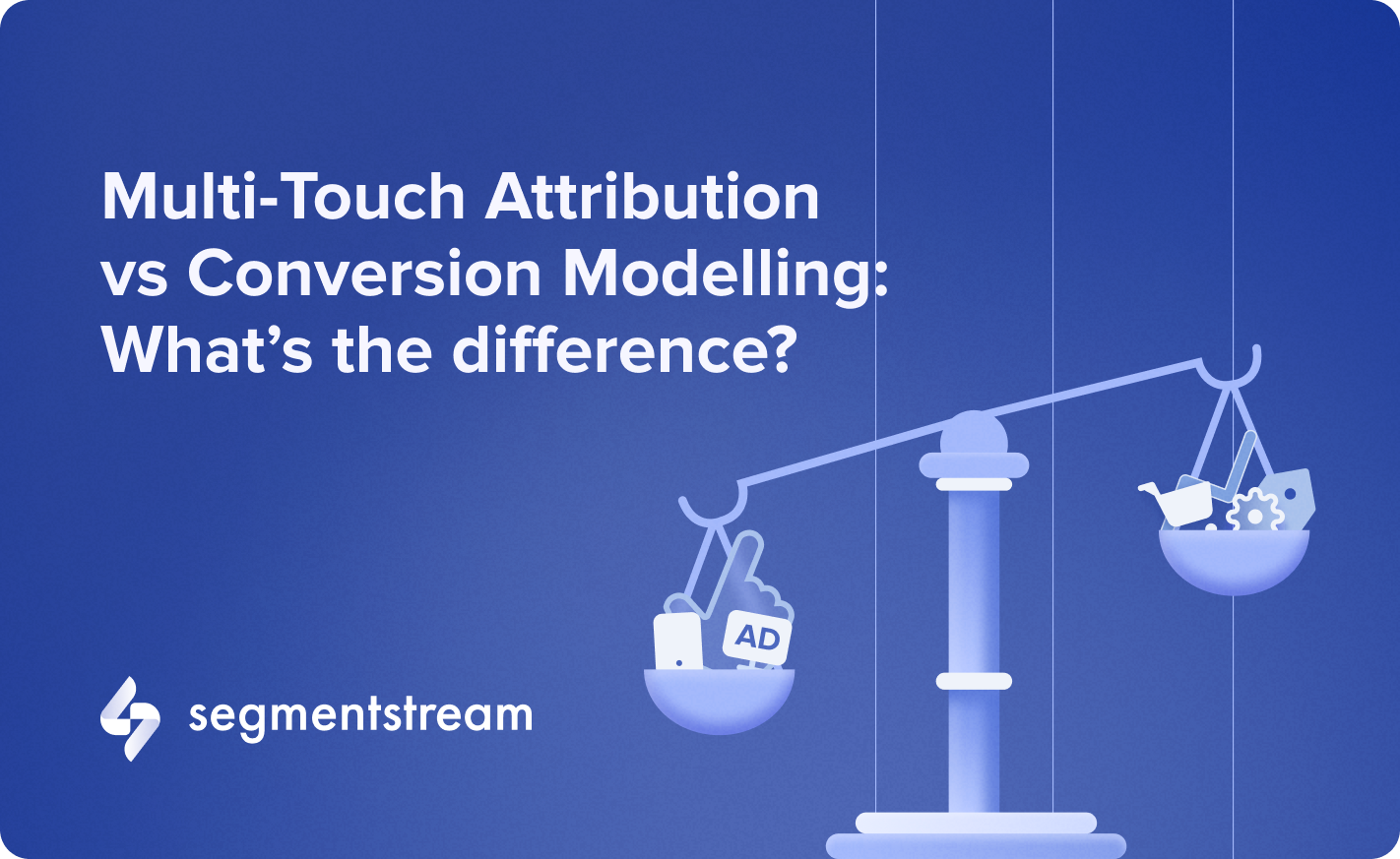
Table of contents
- Agreeing on the definitions
- What these solutions solve
- How much time they need to show actionable results
- How much historical data they need
- How they work with cross-device and cross-browser customer journeys
- Do they work with ITP
- Conclusion
Today’s world offers a wide variety of data-driven measurement and optimization approaches. This makes the life of digital marketers easier and harder at the same time. While Multi-Touch Attribution and Marketing Mix Modelling seem to be the buzzwords for quite some time, the industry evolved with more advanced technologies coming into play.
Which one is the best to solve my business challenges? Do I need to choose just one? How can I decide?
Within this article we’re opening a series of blog posts comparing Conversion Modelling to popular data-driven approaches for performance marketing measurement such as Multi-Touch Attribution (MTA), Marketing Mix Modelling (MMM) and Incrementality Testing.
In this article, we will dive into Multi-Touch Attribution (MTA) and its differences and similarities to SegmentStream.
Let’s agree on the definitions first.
Many measurement and advertising platforms use Single-Touch Attribution models such as Last Click, Last Non-Direct Click or First Click by default. These models will assign all the credit to only one channel from the overall path to conversion, depending on the model used.
Example:
Performance marketing and analytics teams are using Google Analytics Last-Non-Direct-Click approach to understand which channels led to a conversion. They are only able to see channels that serve as the last touch points before the user converted, not taking into account direct/none unless it was the only channel in the path. This approach is heavily biased towards the lower-funnel marketing activities, while the upper-funnel ones actually also play an important role.
Multi-Touch Attribution determines the value of every touch point on the user’s journey to conversion and assigns credit to each of them depending on which attribution model is used. The most common MTA approaches are: Linear, Time-Decay, U-Shape and Data-Driven.
MTA would typically require a certain join key (like a cookie or user login id) to stitch all touch points into a single path.
Example:
Performance marketing and analytics teams are looking to understand the role of each channel within the path to conversion, they’re using Data-Driven MTA to assess their marketing mix performance. This approach could have worked pretty well in the times when users were browsing the internet just from their computers, way before ITP and other privacy solutions were introduced. The challenge is that cookie restrictions, as well as cross-device and cross-browser behaviour limit the ability to track the full journey, meaning many conversions are still getting attributed to the last touchpoint, which often appears to be direct/none. This makes it very hard to trust the data and fairly evaluate the performance of marketing channels.
SegmentStream is an AI-powered platform that enhances marketing budget decisions, offering a reliable alternative to traditional attribution tools.
SegmentStream finds a correlation between ad spend, clicks, website visits (incl. user behaviour) and total sales in the same region/country.
This way, SegmentStream measures true contribution of each channel and campaign on total revenue, even when conversions cannot be directly attributed using cookies.
Okay, so what can these solutions solve for?
Both solutions aim to help with the following use cases:
- High-level Strategy: strategic media planning and channel level budget allocation.
- Tactical Strategy: media planning and budget allocation on campaign level and lower.
- Operational Strategy: digital ads optimization & smart bidding.
Yet, marketers might be struggling to make such decisions based on MTA since it is losing quite a significant amount of data due to complex customer journeys and modern cookie limitations. That’s why many of them switch to SegmentStream for these purposes. We will explain how it is different in more detail in a bit.
How much time would it need to run before I can take action on the results?
MTA would normally require 1-30 days before you can look into the data. That would depend on the attribution window of your business. For example, if your customer’s journey takes 15 days from the first ad click to the purchase, that’s the time you would need to wait before assessing the MTA results, otherwise you won’t see the full picture.
SegmentStream is close to real-time so once the model is up and running you would need around 1 day to start taking actions based on the results.
How much historical data would I need?
MTA would typically need from a few days to a few weeks of data, depending on the algorithm used in the attribution model. For SegmentStream it would also be sufficient to have a few weeks of data so that the ML prediction model can be trained.
Would these approaches account for cross-device and cross-browser customer journeys?
SegmentStream is best suited to solve cross-device and cross-browser challenges since it uses predictive techniques and doesn’t solely rely on user-level tracking. MTA is heavily relying on cookies and thus it’s challenging for it to consider journeys across multiple devices or browsers.
Would these solutions work with the ITP (Intelligent Tracking Prevention)?
ITP has a massive impact on attributing conversions that happen more than 1-7 days after the ad click. SegmentStream would be your best friend to overcome ITP since it doesn’t need to wait for real conversion to happen first. MTA can handle ITP limitations to a small extent, however, it would work only if a conversion happens within 1-7 days after an ad click. If you believe your path to conversion is greater than 7-Days, we suggest you review SegmentStream.
To sum things up,
Although both solutions aim to serve similar purposes, they have different requirements and limitations.
To put it simply, if you’re keen on getting an increased visibility of the true value of your paid media campaigns, along with AI-generated budget allocation recommendations, we suggest you review SegmentStream. MTA can of course still be used, however, it is extremely limited in its ability to handle cross-browser, cross-device, ITP and other cookie limitations. If you’re tired of fighting ITP, complex customer journeys, and are looking for a new way to assess and optimise your Marketing Mix - SegmentStream is something you might want to try.
Optimal marketing
Achieve the most optimal marketing mix with SegmentStream
Talk to expert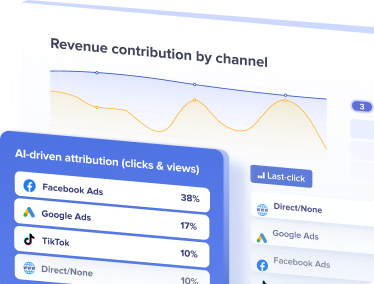