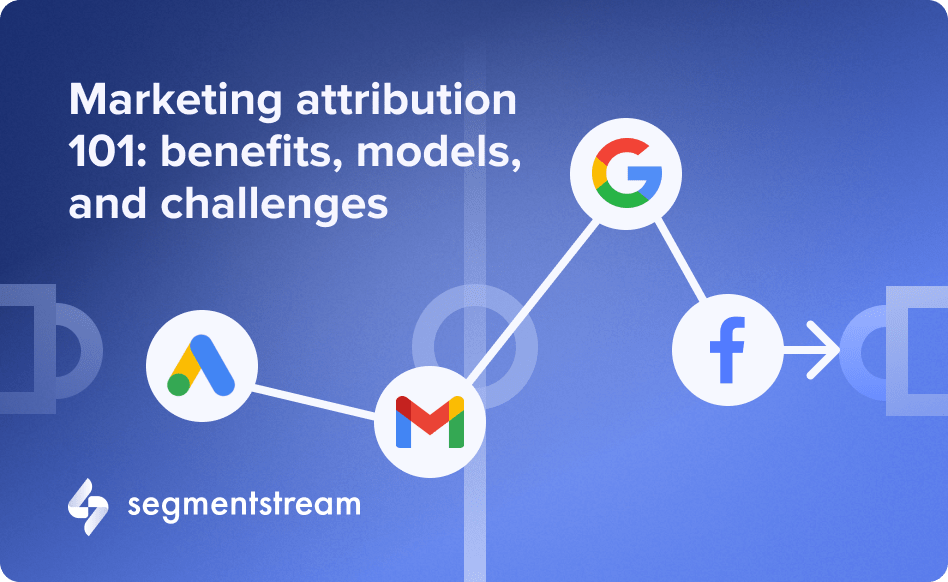
Marketing attribution 101: benefits, models, and challenges — Guide for 2025
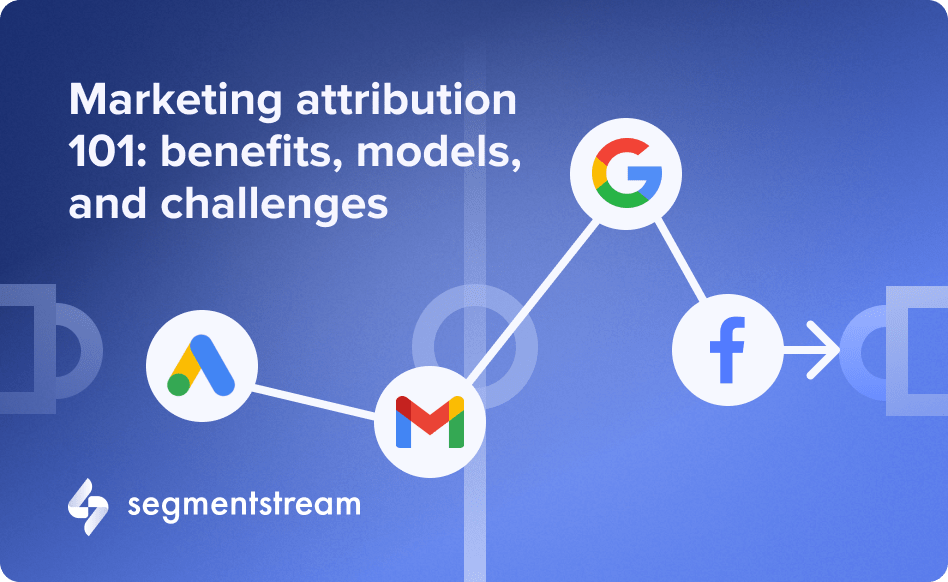
An important skill for every marketing professional is the ability to comprehend which channels are efficiently generating leads and successfully converting customers, along with the reasons behind it.
Precisely forecasting the impact of increased or decreased performance of these channels is essential to execute a sound marketing strategy. However, with multiple engagement points and increasingly complex buyers’ journeys, it has become more challenging for marketers to attain this level of visibility.
Casually running reports on isolated channels, such as web traffic or email open rates, can’t help with developing a reliable marketing engine. Unless marketers know how these channels are affecting their business outcomes, these reports are nothing but superficial metrics.
Gaining visibility into this type of information for marketers can look like sifting through disparate data from various sources, or spending several hours crunching numbers in spreadsheets unless they have access to marketing attribution tools.
In this article, we will dig deeper into the following topics:
- What is marketing attribution?
- How does marketing attribution work?
- What typical challenges do the attribution models face?
- How to overcome the challenges with marketing attribution?
What is marketing attribution?
Marketing attribution is the process of identifying and assigning credit to the marketing channels that lead to a conversion or sale. It helps businesses to understand which marketing channels are most and less effective in driving leads and sales.
There are several marketing attribution models that assign credit to one or more touchpoints in the customer journey following particular rules, which we will also review later.
First, let’s take a look at the benefits that digital marketing attribution brings.
Marketing attribution benefits
Here are some of the most significant benefits that businesses get from using marketing attribution:
Optimisation of marketing spending
By identifying which channels and touchpoints are driving conversions, businesses can allocate their marketing budget more efficiently. They can invest more in the channels and campaigns that are generating the highest return and reduce spending on those that are less effective. This not only helps to optimise the budget but also results in a better return on investment (ROI).
Increasing ROI
Marketing attribution helps businesses to understand the customer journey and the touchpoints that lead to conversions. By knowing which channels and marketing activities are most effective, companies can create better-optimised campaigns that resonate with their target audience. This can increase the chances of converting leads into customers and improve ROI.
Improves ad platform performance
Marketing attribution can provide feedback signals to ad platforms, such as Google Ads and Facebook Ads, about how different advertising channels work. These ad platforms use complex algorithms to optimise ad delivery and targeting to reach the right audience at the right time, and feedback signals from marketing attribution can help improve the performance of these algorithms.
In particular, the ad platforms use Smart Bidding algorithms built with Machine Learning to determine how to allocate ad spend and adjust bids for individual auctions in real time. These algorithms take into account a range of factors, such as device type, audience behaviour, and historical performance data to determine the optimal bid for each auction.
The feedback signals provided by marketing attribution can also help ad platforms identify areas where their algorithms can be improved. By analysing attribution data, ad platforms can identify trends and patterns in customer behaviour and adjust their algorithms to better predict customer intent.
In summary, marketing attribution is a powerful tool that can help businesses optimise their marketing spending, increase their ROI, and provide valuable feedback to advertising platforms. By using marketing attribution, businesses can improve the effectiveness of their marketing campaigns and ultimately drive more conversions.
There are various digital marketing attribution models that can be used for different purposes. Let’s make an overview to understand how and when to use them.
Marketing attribution models
Overall, all marketing attribution models can be divided into two major groups: single-touch attribution models and multi-touch attribution models.
Single-touch attribution models
These models give credit for a conversion to a single touchpoint or channel. They are often simpler to implement, but they are less accurate in attributing credit to the touchpoints that actually led to a conversion.
First touch attribution
In this model, credit is given to the first touchpoint a user had with a business. For example, if someone clicks on a Facebook ad, and then later converts through an email campaign, the credit goes to Facebook.
It does not give credit to any other marketing interactions that might have played a role in the customer’s decision to purchase.
Last touch attribution
In this model, credit is given to the last touchpoint a user had with a business. This model is useful in tracking the effectiveness of the last touchpoint before the sale, such as a retargeting ad or email campaign. It overlooks the role that previous touchpoints might have played in nurturing the customer towards the sale.
For example, if someone clicks on an organic search result, and then later converts through a paid search ad, the credit goes to paid search.
Last non-direct click attribution
In this model, credit is given to the last touchpoint a user had with a business that was not a direct visit to the website. Direct traffic is typically excluded because it can be difficult to determine the source of the traffic.
For example, if someone clicks on a display ad, and then later converts through an email campaign, the credit goes to display.
Last non-brand click attribution
This model gives all the credit to the last marketing interaction which was a non-branded search term or ad. Brand searches are those where the user searches for the company name or a related brand term.
This model helps to identify the effectiveness of non-branded campaigns in driving conversions, but it may not capture the full value of brand awareness campaigns.
Last paid click attribution
This model attributes all the credit for a conversion to the last paid marketing channel, which could be a paid search ad, display ad, social media ad, or any other paid marketing channel that the customer interacted with before converting.
For example, suppose a customer clicks on a paid search ad, then clicks on a social media ad, and finally makes a purchase. In that case, the last paid click attribution model would give all the credit for the sale to the social media ad.
Multi-touch attribution models
Multi-touch attribution models give credit for a conversion to multiple touchpoints or channels. Such models can be more complex to implement, but they can provide a more accurate picture of which touchpoints contributed to a conversion.
Linear attribution
In this model, credit is distributed evenly across all touchpoints that led to a conversion. For example, if a user sees a Facebook ad, clicks on an organic search result, and then converts, each touchpoint receives equal credit.
Time decay attribution
In this model, more credit is given to touchpoints that are closer in time to the conversion. For example, if a user sees a display ad, clicks on a paid search ad, and then converts, the paid search ad receives more credit because it was closer in time to the conversion.
U-shaped attribution
This model gives equal weight to the first and last touchpoints in the customer journey: 40% of the credit for a conversion is given to the first touchpoint and another 40% is given to the last touchpoint. The remaining 20% is divided equally among all other touchpoints in the customer journey.
For example, suppose a customer first discovers a brand through a social media ad, then later clicks on an email campaign and finally makes a purchase after clicking on a search ad. In the U-shaped model, the social media ad and search ad would each receive 40% of the credit, while the email campaign would receive 10%. The remaining 10% would be divided equally among all other touchpoints.
Data-driven attribution
The model typically involves analyzing data from various marketing channels such as social media, email marketing, search engine marketing, and display advertising. The data is then used to identify the touchpoints that customers interacted with before making a purchase or taking a desired action.
Once the touchpoints have been identified, the model assigns a weight to each touchpoint based on its contribution to the final conversion. The weight is determined using a statistical algorithm that takes into account various factors such as the timing and frequency of the touchpoints.
Algorithmic attribution
In this model, a machine learning algorithm is used to determine the credit assigned to each touchpoint based on historical conversion data. The algorithm may take into account factors such as:
- the type of touchpoint,
- the position of the touchpoint in the customer journey,
- and the time since the touchpoint occurred.
This model can be more accurate, but it requires a significant amount of data to train the algorithm.
It’s important to note that there is no one “right” marketing attribution model. The best model for a business will depend on its unique goals, customer journey, and marketing mix. It’s often a good idea to test different models to see which one provides the most useful insights.
Also, it’s important to be aware of the common challenges with digital marketing attribution, such as cross-device and cross-browser journeys, long sales cycles, lack of proper tools and applications, and data regulations and tracking restrictions. Addressing these challenges can help to ensure that attribution in digital marketing is as accurate and effective as possible.
Common challenges with marketing attribution models
While marketing attribution is a powerful tool, it comes with a few common challenges that you should be aware of:
Cross-device and cross-browser journeys
It can be challenging to accurately track customer journeys that span multiple devices and browsers. This can lead to discrepancies in attribution results and underreporting of the impact of certain channels.
For example, if one customer interacts with an ad on their mobile device but makes a final purchase from their home laptop via a direct visit to the website, the initial touch channel won’t get any credit at all.
Long sales cycles
When the customer journey is long and complex, it can be difficult to attribute conversions to a specific channel or campaign accurately. The longer the sales cycle, the higher the possibility that the conversion won’t happen within the same cookie.
This can lead to inaccuracies in attribution results and make it challenging to optimise your marketing spend effectively.
Inability to calculate ROAS and ROI properly
Accurately measuring the ROI of your marketing efforts can be challenging, especially when considering the long-term impact of campaigns or channels. This can lead to difficulty in determining which channels and campaigns are driving the highest ROI and making it harder to optimise your marketing spend.
As marketers face increasing restrictions on tracking user behaviour with cookies, calculating the return on advertising spend (ROAS) for each channel becomes nearly impossible. Attempting to measure ROAS at a granular level for every campaign and channel is a futile exercise that can lead to improper optimisation of the marketing mix itself.
Lack of proper tools and applications
To implement attribution in digital marketing effectively, you need the right tools and applications to track customer journeys and analyse data. Most of the tools and attribution models rely on cookies heavily, which is not the best strategy today.
The cookies are becoming less reliable as a tracking mechanism because of growing privacy concerns — many users are now opting out of being tracked. In addition, various web browsers, including Safari, Firefox, and Chrome, are blocking third-party cookies by default, which limits the effectiveness of traditional attribution methods. Finally, new regulations like GDPR and CCPA have imposed strict rules on how cookies can be used, which has further reduced their effectiveness as a tracking mechanism.
As a result, marketers are facing challenges in accurately tracking user behaviour and attributing conversions to the right channels. To overcome this challenge, marketers need to explore alternative tracking mechanisms that are privacy-safe and provide reliable attribution data.
The rise of the cookieless world
In a cookieless world, it becomes more difficult to track a user’s journey across multiple touchpoints and accurately attribute credit to each one.
Without cookies, marketers may need to rely on other tracking technologies such as device fingerprinting or server-side tracking, which may not be as accurate or reliable as cookies. Additionally, aforementioned privacy regulations restrict the use of cookies and other tracking technologies, making it more difficult to collect user data and attribute conversions to specific touchpoints.
As a result, even multi-touch attribution models deliver results similar to single-touch ones. This is why marketers are constantly looking for ways to overcome those issues, and are in great need of an alternative tool that can actually work in a cookieless world.
How to solve the challenges with attribution in digital marketing?
With traditional marketing attribution methods struggling in today’s digital environment, AI-driven solutions like SegmentStream are offering more effective alternatives.
SegmentStream differentiates itself from conventional models by evaluating a wide array of data, including user behavior, without relying heavily on cookies or tracking the entire customer journey. This approach provides a more accurate and privacy-conscious assessment of marketing impacts.
Core Solutions of SegmentStream:
-
Incremental Attribution:
- The platform measures incremental ROAS across digital campaigns by analyzing user behavior, reducing its reliance on cookies. This method gives a clearer picture of how each campaign influences sales. More about Incremental Attribution.
-
AI-Driven Marketing Mix Optimization:
- SegmentStream uses AI to offer strategic budget recommendations, aiming for an effective allocation of marketing resources. This tool aids in determining the most efficient use of budget across different channels. Explore Marketing Mix Optimization.
Overcoming Modern Challenges
By using first-party data and machine learning, SegmentStream goes beyond traditional cookie-dependent methods, aligning well with the increased focus on privacy in digital marketing. Its advanced solutions are designed to meet the specific needs of modern marketers, providing insightful analysis and aiding in the optimization of marketing strategies.
To sum up
Marketing attribution models help to analyse the customer journey and optimise marketing campaigns for maximum impact.
In this guide, we explored the different types of marketing attribution models, including single-touch and multi-touch ones, discussed their benefits, and explored the typical challenges associated with attribution modelling, including the impact of the cookieless world.
In conclusion, marketing attribution models are a powerful tool for businesses looking to optimise their marketing campaigns and drive more conversions. Yet, they are highly impacted by all tracking restrictions and privacy regulations.
To deal with these challenges, marketers turn to alternative solutions such as SegmentStream’s platform.
It enhances marketing budget decisions, offering a reliable alternative to traditional attribution tools. And unlike traditional cookie-based solutions offers a privacy-focused approach, aligning with the latest industry standards.
Optimal marketing
Achieve the most optimal marketing mix with SegmentStream
Talk to expert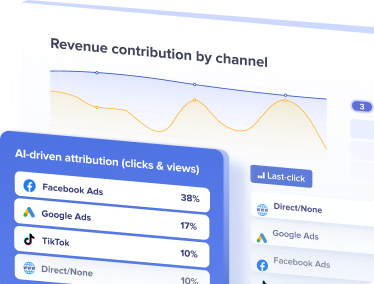