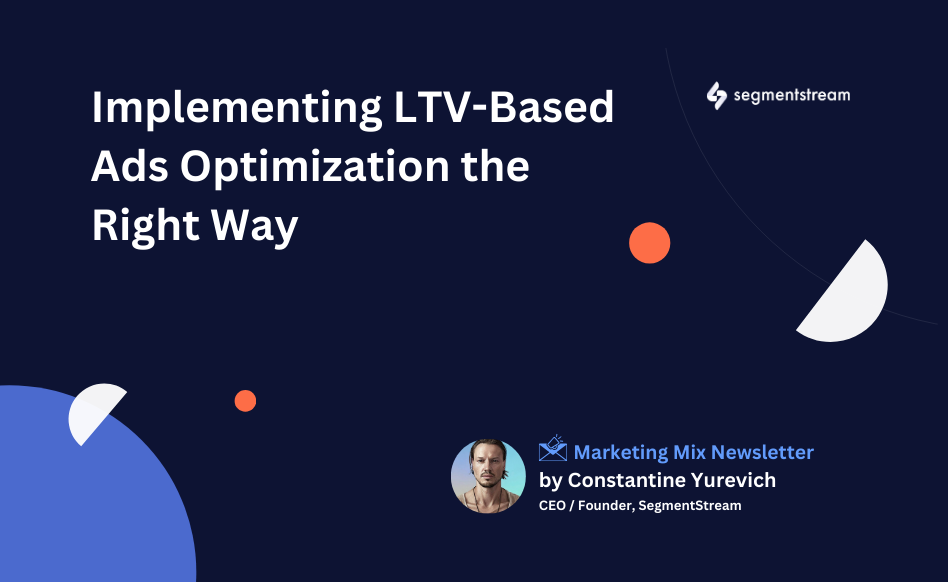
Implementing LTV-Based Ads Optimization the Right Way
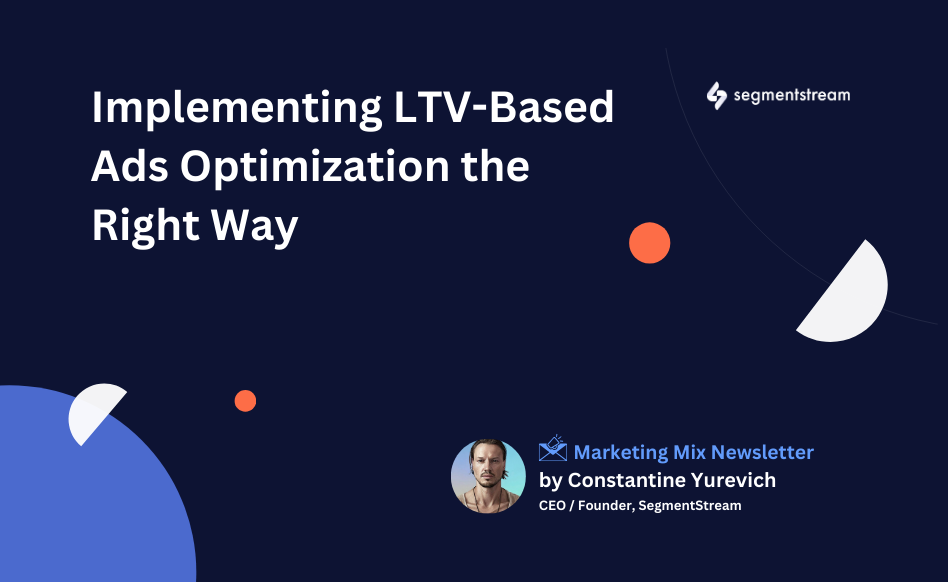
Optimiziation towards LTV is one of the most challenging tasks you could do. Even though, for some types of businesses this is the only way to make a robust and profitable future-looking customer acquisition that will guarantee sustainable growth.
Businesses that might be interested in LTV-based optimization include:
- DTC brands with lots of repeating orders (apparel, gift cards, books, etc.)
- SaaS businesses
- Other subscription-based services
- Mobile apps (games or subscription-based apps)
- Gambling
- etc.
LTV optimization is a complex task and there are so many marketers that approach it in a completely wrong way.
Common Mistakes in LTV Optimization
1. Calculating Average LTV
Using a simple average can be misleading. Different user segments have widely varying lifetime values. By averaging these together, you risk overpaying for segments that bring lower returns while underinvesting in those with high LTV potential. It’s essential to segment your customers accurately and tailor strategies for each group.
2. Calculating LTV at the Channel Level
Statements like “My LTV from TikTok is lower than from Facebook” are fundamentally flawed. LTV is about understanding the user—their traits, behaviors, and lifecycle—not merely the acquisition channel. For example, a predominantly younger audience on TikTok might prefer monthly plans, whereas older users from Facebook might lean towards annual subscriptions. Comparing these channels directly is akin to comparing apples to oranges; it’s the user’s profile that truly matters.
3. Incorrect LTV Calculation Methods
Errors in applying the LTV formula can lead to inconsistent results across different user cohorts. LTV is inherently a cohort metric. For many businesses, starting with a 1-year LTV can provide a consistent benchmark. This approach involves analyzing all signups over the past 3-4 years and measuring their revenue contribution within the first year. Attempting to calculate a true “lifetime” LTV without considering customer age or lifecycle stage can result in volatile and unreliable figures.
4. Neglecting LTV Forecasting
Even with a 1-year LTV framework, the real value of your customers is only visible with a significant delay—typically a year. This lag renders traditional LTV calculations impractical for real-time analytics and ad optimization. Implementing real-time LTV forecasting enables businesses to immediately assess new customers, understand their Customer Acquisition Cost (CAC), and adjust advertising strategies on the fly. This dynamic feedback loop is essential for agile decision-making in fast-paced markets.
Real-World Application: Optimizing LTV for a Complex SaaS Business
Let’s consider a SaaS business that operates on a freemium model and offers multiple pricing plans. This business has a variety of conversion points:
- Freemium registration
- Basic Monthly plan at $50/month
- Basic Annual plan at $500/year
- Standard Monthly plan at $200/month
- Standard Annual plan at $2000/year
- Booked demo, which may convert in 4–7 months into a large enterprise deal worth between $50k to $150k/year
In addition, users may upgrade or downgrade their plans over time, making the customer journey highly dynamic and complex. With such a multifaceted revenue structure, the marketing department’s goal of “Driving more LTV” becomes especially challenging. Critical questions emerge, such as:
- Should the focus be on monthly subscriptions or annual commitments?
- How much should be invested in acquiring freemium users versus paying for monthly or annual conversions?
- What is the accurate valuation of booked demos, given their potential to evolve into high-value enterprise deals?
Instead of exploring all the flawed approaches that often complicate this analysis, we’ll cut straight to how we tackle these challenges at SegmentStream.
Implementation: A Step-by-Step Guide
Step 1: Establish the Normalization Period
Decide on the normalization period of your LTV. Remember, LTV is a cohort metric. Even for a 1-year LTV, you must only include users whose first sign-up occurred at least one year ago; otherwise, your LTV will be biased and undervalued. This rule applies even more so when considering 2-year or 3-year LTV calculations. As a rule of thumb, we typically recommend using a 6- to 12-month cohort to balance customer value with data recency and market dynamics—especially when LTV is used for marketing optimization. For this article, let’s assume we’ve chosen a 1-year normalized LTV.
Step 2: Identify the Key Segments
Calculating an average LTV might seem reasonable with a small number of conversions, but it’s often misleading. The next step is to determine which user parameters most influence LTV and to build segments accordingly. For a global startup with various subscription plans like those mentioned, two properties usually work well:
- User Country Tier: Instead of using all 195 countries, we group them into tiers (e.g., Tier 1, Tier 2, Tier 3).
- Initial Subscription Plan (First Sign-Up Context): Options include: (Freemium registration, Basic Monthly plan at $50/month, Basic Annual plan at $500/year, Standard Monthly plan at $200/month, Standard Annual plan at $2000/year, Booked demo)
Combining these factors already creates 18 segments(!!!). This number is often sufficient—each segment must have enough conversions to ensure accurate predictions and avoid overfitting.
But of course sometimes we go more granular with other segments like:
- Relevant role of the buyer (yes/no)
- Business email vs personal (yes/no)
- etc… but let’s keep it outside of the scope of this article
Step 3: Calculate Segment-Based LTV
For each segment, use data from users who signed up at least one year ago to calculate the 1-year LTV. For instance:
- What is the average revenue from a Tier 1 user who started with a Freemium registration over their first year?
- What is the average revenue from a Tier 3 user who booked a demo over their first year?
Repeat this process for all 18 segments to capture the diverse revenue profiles across different user groups.
Step 4: Integrate LTV Forecasting into Real-Time Analytics
Traditionally, you would wait at least one year to know the LTV of a signup. With a robust LTV-forecasting model built from historical data (as in Step 3), you can forecast LTV in real time.
For example, if a new Tier 1 user signs up for the Basic Monthly plan at $50/month, you simply input two variables—pricing plan (Basic Monthly) and country tier (Tier 1)—into the model. The model might immediately forecast a 1-year LTV of $400, which is then attributed to the channel that acquired the user, rather than just the $50 from the first month.
This process applies uniformly to all sign-ups—be they Freemium, Monthly, Annual, or even Booked Demos for Enterprise deals—transforming your analytics into a 1-year cohort LTV framework that drives informed budget allocation decisions.
Step 5: Feed Forecasted LTV Signals into Ad Platforms
This is the step many advertisers overlook.
Ad platforms are highly sensitive to data recency; some (like Facebook Ads) might reject signals older than 7 days. Previously, waiting one year rendered LTV data too stale for real-time bidding.
Now, with a real-time forecasting model in place, you can immediately send forecasted LTV values to your ad platforms. For example, when a Tier 1 user signs up for the $50/month Basic plan, you instantly send a forecasted LTV of $400 using the Conversions API. Simultaneously, it’s crucial to switch your bidding strategy from conversion-based (e.g., Target CPA or Maximize Conversions) to value-based bidding (e.g., Target ROAS or Maximize Conversion Value).
This dynamic, automated system prioritizes users with higher forecasted LTV and adjusts bids accordingly—no manual adjustments or custom rules necessary.
Conclusion
There are countless nuances not covered in this conceptual article, such as:
- Building a validation set to assess prediction quality.
- Automating the model to continuously incorporate new data and maintain a sliding 1-year cohort.
- Calculating Marginal ROAS to account for diminishing returns.
- Implementing attribution methods that extend beyond last-click analysis.
All these aspects are fully automated within the SegmentStream platform, allowing you to have such a model up and running in a very short term. Implementing LTV forecasting can be a game-changer for any business model focused on more than just the first purchase, streamlining your revenue growth exponentially over the long term.
Hope this guide proves valuable as you implement a robust, LTV-based optimization strategy — one that sidesteps the common pitfalls discussed earlier.
Optimal marketing
Achieve the most optimal marketing mix with SegmentStream
Talk to expert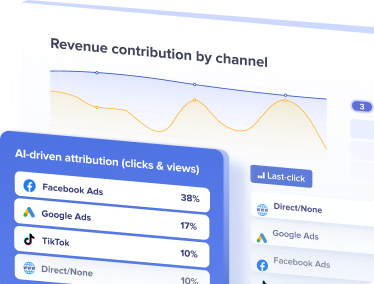